Computer Blood Sugar and Tracking A1c Software
ABSTRACT
It is recognized that traditional measures of glucose control (such as hemoglobin A1c [A1C]) provide little information regarding the need for day to day changes in therapies. While intermittent self-monitored blood glucose (SMBG) provides additional information with which to make treatment decisions, significant barriers to its use exist, such as inconvenience and lack of timely and regular feedback. Furthermore, important information regarding glucose trends may be missed. Continuous glucose monitoring (CGM) has become increasingly reliable and has demonstrated efficacy in terms of improving A1C, reducing hypoglycemia, and improving the time in target glucose range. Incremental progress continues to be made toward a fully functional artificial pancreas, of which CGM will play a vital role. As more and more data are presented to patients and providers, it has become increasingly paramount that the data are organized in a standardized way and that communication of data is streamlined using patients' mobile devices where available and within the existing clinic infrastructure. Systems that provide immediate feedback to patients and decision support tools for patients and providers have demonstrated superior outcomes compared to routine SMBG alone. Alternate markers of glucose control may provide complementary information about glucose control and long-term prognosis. This chapter will review the latest evidence for use of professional and personal CGM, mobile glucose monitoring approaches, and biomarkers of glycemic control. For complete coverage of all related areas of Endocrinology, please visit our on-line FREE web-text, WWW.ENDOTEXT.ORG.
INTRODUCTION
The current technology for monitoring of glucose levels has been well established since the 1980′s. This practice is beneficial to patients with diabetes from both a clinical and an economic standpoint when used optimally. Knowledge of the glucose levels that are measured can allow a patient to select an appropriate dose of insulin or implement dietary or other lifestyle changes to regulate their glucose levels. Expert groups provide recommendations for glucose targets, including HbA1c, self-monitored blood glucose (SMBG), and interstitial glucose (1,2). Although targets vary, expert groups recommend individualization based upon risk of hypoglycemia, polypharmacy, comorbidities, and other characteristics that may affect long-term benefit and individual patient characteristics.
The landscape of glucose monitoring technologies is expanding and rapidly changing. For a full review of glucose monitoring technologies, the reader is referred to one of many excellent reviews referenced throughout this chapter. In addition, Diabetes Forecast publishes an up to date description of glucometers, continuous glucose monitoring (CGM) devices, diabetes apps, and their features regularly (3). Several trends are emerging in glucose monitoring and will be reviewed in more detail in this chapter:
-
CGM: This practice is becoming more widely established as evidence supporting its use has accumulated. The data available through CGM can permit significantly more fine-tuned adjustments in insulin dosing and other therapies than spot testing from self-monitoring of blood glucose (SMBG) can provide. CGM technologies for automatic collection of data have spurred interest in noninvasive glucose monitoring as an additional tool for obtaining information about glucose levels.
-
Closed loop control (CLC): Also known as an "artificial" or "bionic" pancreas, this technology links CGM with automatically controlled insulin delivery. The first steps toward CLC are now in use.
-
Mobile Technology and Decision Support: In recent years, increasing connectivity between glucose monitoring technologies and mobile devices has facilitated ongoing improvements in self-care and communication of data.
-
Alternate Markers of Glucose Control: Finally, the use of additional analytes besides glucose is still being established.
This chapter analyzes the technology, benefits, and problems with the use of intermittent SMBG and CGM, mobile technology and decision support, and alternate biomarkers of glycemic control.
CONTINUOUS GLUCOSE MONITORS
CGM measures glucose levels (typically interstitial glucose) continuously and updates the glucose level display every 1 to 5 minutes. Most CGMs consist of 1) a monitor to display the information (in some cases, this is the patient's mobile device), 2) a sensor that is usually inserted into the subcutaneous tissue, and 3) a transmitter that transmits the sensor data to the monitor. Previously, all devices were approved for adjunctive use only due to limitations in accuracy; in this case patients must still perform fingerstick glucose monitoring in order to guide therapy and perform calibrations. However, in 2016, the FDA approved the use of the Dexcom G5 as the first CGM for stand-alone use. Newer technologies have eliminated the requirements for calibration of CGM with a fingerstick glucose. The accuracy of all commercially available CGMs is still the lowest in the hypoglycemic range, which is where the need for sensitivity and specificity is great in terms of serving as an alarm for hypoglycemia.
CGM can provide both retrospective as well as real-time information to detect: 1) hypoglycemic and hyperglycemic excursions; 2) predict impending hypoglycemia; and 3) wide fluctuations in glucose levels, also known as glycemic variability. 24-hour telephone support is available for all FDA approved CGM devices. Use of CGM can help both the patient and their medical provider make fine tune adjustments to medication therapy and provide insight to the patient on behavioral changes to achieve glycemic control. Additionally, current efforts to link CGM measurement with automatically controlled insulin delivery, has progressed incrementally toward a fully functional artificial pancreas. Systems can be divided according to their intended use as professional CGM (which is a clinic-owned device and provides either retrospective or real-time glucose data) and personal CGM (which is patient-owned and provides real-time glucose data).
PROFESSIONAL CGM
Professional CGM describes CGM data that are obtained via healthcare provider owned equipment. It does not necessarily provide the glucose results in real time, but downloads the readings after they have been collected, similar to a 24-hour cardiac Holter monitor that provides information about cardiac rhythms after they have occurred. This allows the health care provider to obtain relatively unbiased glucose patterns during typical everyday life. The Endocrine Society recommendations state that professional CGM may be of benefit in adults with diabetes to detect nocturnal hypoglycemia, dawn phenomenon, postprandial hyperglycemia and to assist in management of diabetes therapies (4). Professional CGM is more readily reimbursed than personal CGM, but interpretation of both personal and professional CGM reports by qualified healthcare professionals may be reimbursed on a monthly basis.
Some personal CGM systems can be operated in a blinded fashion in order to provide professional glucose data. These systems will be discussed in more detail later (see "Personal [Real-time] Continuous Glucose Monitoring"). The first device for reading blood glucose levels continuously was a professional CGM that was approved by the FDA in June 1999. This device was the Continuous Glucose Monitor System (CGMS) manufactured by Medtronic MiniMed (Medtronic Diabetes, Northridge, CA) (5). Since then, newer models have shown improvements in accuracy and patient acceptance.
iPro2
The iPro2 was approved by the FDA in 2011 (Figure 1) (6). The device calculates and stores glucose readings every five minutes. The iPro2 uses the Enlite sensor, which contains a wire with a supply of glucose oxidase at the tip which is inserted subcutaneously into the anterior abdominal wall with a dedicated inserting device, the Enlite Serter. The same enzyme for recognizing glucose molecules is used in many portable blood glucose monitors. Glucose oxidase catalyzes a biochemical reaction in the presence of glucose and oxygen that transfers electrons to a receiving molecule and creates an electronic current, whose magnitude can be measured and converted into a glucose concentration. After up to 6 days of measurements, the device is removed and plugged into a docking station to download its readings into a computer.
The iPro2 is cordless and requires minimal patient interaction or training. It is activated, inserted, and downloaded in a few simple steps. All calibrations of the iPro2 are performed at the time the device is downloaded by the healthcare provider, either via downloading compatible meters or manual entry. The sensor is identical to that used in the Medtronic Guardian Realtime CGM and Paradigm Revel sensor augmented pump. However, both retrospective and prospective time-points are available for calibration with the iPro2, potentially improving overall accuracy compared to real-time CGM. The mean absolute relative difference (MARD) is reported to be 9.9-10.1% overall and lower sensor-meter agreement in the hypoglycemic range (40-80 mg/dl) (7). The docking station can be connected to a computer that contains dedicated software, called Carelink iPro Software, for use with the system. Patients can also use the iPro2 myLog App to log events that might be useful for interpretation of the data, such as blood glucose levels, diet, and activity. These data are automatically integrated within the CareLink Pattern Snapshot report, which provides summary glucose statistics, percentage of time spent within, above, and below target range, and patterns.
Figure 1.
iPro2 Continuous Glucose Monitor. A –Monitor. B – Docking station.
FreeStyle Libre Pro
The FreeStyle Libre Pro utilizes the same sensor as the Libre personal CGM. Unlike the iPro2, the Libre is factory calibrated and therefore does not require self-monitored blood glucose calibrations. This may be a potential advantage since capillary blood glucose testing is subject to various system and user errors, which in addition to the physiologic lag time between blood and interstitial glucose (which is magnified in the postprandial period) could contribute to CGM error. It collects up to 14 days of glucose readings, which are recorded every 15 minutes. The glucose sensor is fully disposable and a single reader is used to activate and scan multiple devices, allowing multiple patients in one office to undergo the procedure simultaneously.
Reports are obtained through the LibreView website, which offers a secure cloud-based system, or the FreeStyle Libre desktop reporting software. Reports provide daily patterns, an assessment of glucose variability and hypoglycemia risk, a daily glucose report, and an overall snapshot report.
The overall MARD for the FreeStyle Libre is 11.4%, 86.7% of readings were in Zone A of the Consensus Error Grid analysis, and 99.7% of results were in Zones A and B.(8) It is important to note that sensor accuracy is lower on day 1 and in the hypoglycemia range (MARD 20.3% for values <72 mg/dl in one study) (9,10). Accuracy improves and remains steady over the 14-day wear period. The Libre utilizes glucose oxidase in a "direct signaling" approach that is not dependent on oxygen and minimizes interference by other substances, such as acetaminophen, which may falsely elevated readings on other devices.
Dexcom Professional
The Dexcom G4 PLATINUM Professional CGM is a practice-owned system that offers real-time or blinded data depending upon whether the goal is to observe glucose patterns without intervention, to provide immediate feedback to educate and inform patients about their medications and behaviors, or to facilitate decisions about pursuing personal CGM. Patients are required to perform fingerstick calibration of the device twice daily when wearing the CGM. The sensor, transmitter, and receiver are essentially identical to the personal Dexcom G4 system.
The device measures interstitial glucose levels every 5 minutes and is approved for 7 days of use. The device is downloaded using Dexcom CLARITY, a web-based software program that is also used to download and review personal data.
The Dexcom G6 Pro was approved by the FDA in March 2018 and is available in blinded or unblinded mode. It features 10-day sensor wear time, expedited startup time, and no calibration.
Analysis of Retrospective Data
Data from all CGM devices can be studied retrospectively after downloading (11). It is recommended that diet, activity, symptom, and insulin data are collected during professional CGM to assist with interpretation, either via patient diary, direct entry of events into the device, or use of an accompanying app, depending on the system. Three time periods should be analyzed. These are:
-
Overnight: Out-of-target overnight glucose levels can be modified by adjusting the basal insulin dose.
-
Pre-prandial Period: Out-of-target pre-prandial glucose levels can be modified by adjusting the previous meal bolus, meal, or exercise pattern.
-
Post-prandial period: Out-of-target postprandial glucose levels can be modified by adjusting the immediate meal bolus, meal, or exercise pattern.
In certain special situations, targets may need to be adjusted. Other important elements of a professional CGM analysis are shown in Table 1. An example of a patient who used CGM (with iPro2) is presented in Figure 2. The CGM demonstrated high glucose levels from 6:00 PM to 11:00 PM post-supper and low glucose levels from 12:00 AM to 2AM. Recognition of these patterns allowed appropriately timed treatment interventions.
Table 1.
Elements of Professional Continuous Glucose Monitoring Analysis
View in own window
Overall Control
Mean Glucose
Glucose Variability (Standard Deviation, Coefficient of Variation)
Daily Detail
Diurnal Patterns: dawn phenomenon, overnight Meal effects
Correction Exercise effects
Other patterns (work days vs. weekend, menstrual cycles)
Hypoglycemia
Precipitating factors
Corresponding meter glucose (recognition)
Figure 2.
iPro2 tracing of a patient whose glucose levels were high from 6:00 PM to 11:PM post-supper and low from 12:00 AM to 2AM.
Ambulatory Glucose Profile
The ambulatory glucose profile (AGP, Figure 3) is a standardized reporting format for glucose data that was developed by an expert panel of diabetes specialists and sponsored by the Helmsley Charitable Trust and is customized for insulin pumps or injection therapy (12). The universal report is intended to simplify and facilitate interpretation of otherwise complex and lengthy reports with varying terminology. It is anticipated that a standardized report would "help clinicians develop expertise in CGM use, enhance quality of care through enhanced pattern recognition, improve practice efficiencies with minimal disruption of workflow, and engage patients, thereby reinforcing consistent use of CGM technology." A single page report that the medical team can view and file into a patient's electronic medical record and that can be used as a shared decision-making tool with people with diabetes was considered to be of great value in the report of the 12th International Conference on Advanced Technologies & Treatments for Diabetes (ATTD 2019) (13). The AGP is currently employed by many reporting systems and consists of 3 components:
- 1.
-
Statistical Summary, which utilizes standard metrics and terminology to summarize the number of values, percentage of values and time in target, above target, and below target, as well as an assessment of glucose variability.
- 2.
-
Modal day report which collapses data from days or weeks to a single day in order to identify patterns by time of day. Data are presented graphically as 5 distribution curves, representing the median, interquartile range, and 10th to 90th percentiles, on the backdrop of target range.
- 3.
-
Daily View, which facilitates review of within day events.
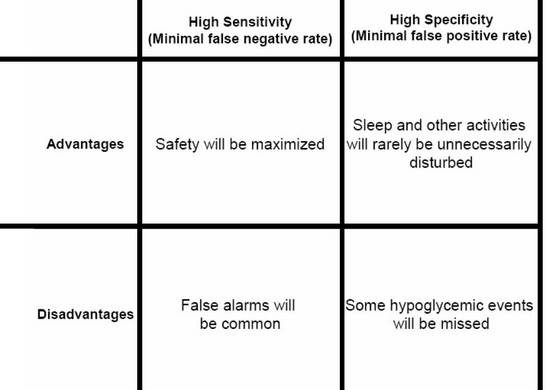
Figure 3.
Ambulatory Glucose Profile for Insulin Pumps.
Glucose Statistics: Metrics include mean glucose, estimated A1C, glucose ranges, coefficient of variation and standard deviation.
Glucose Profile: Daily glucose profiles are combined to make a one-day (24-hour) picture. Ideally, lines would stay within grey shaded area (target range)
Orange: median (middle) glucose line
Blue: area between blue lines shows 50% of the glucose values
Green: 10% of values are above (90% top line) and 10% are below (10% bottom line) Insulin Profile Graph: Shows basal insulin pump settings over a 24-hour period
Bolus Insulin Graph: Combines all bolus insulin doses into one graph to make a one day (24-hour) picture. Each box on the graph covers 60 minutes of doses.
Orange: median (middle) dot
Blue: shaded box shows 50% of the bolus dosages in the hour
Green: lines above and below the shaded box (whiskers) show how many of the bolus dosages per hour were between 75 - 90% and between 10 - 25%
PERSONAL (REAL-TIME) CONTINUOUS GLUCOSE MONITORING (RT-CGM)
RT-CGM devices not only display the current glucose every few minutes, but may also alert the patient for impending (projected alert) or actual (threshold alert) hyperglycemia or hypoglycemia or rate of change in glucose. Over time, accuracy with RT-CGM has improved substantially (14,15,16). In fact, several devices, the Dexcom, Freestyle Libre, and Eversense are approved for stand-alone use, meaning that under specified conditions, the device may be used to make treatment decisions without confirmatory blood glucose measure. However, the user will still experience a tradeoff between a high alarm sensitivity and specificity for detecting hypoglycemic events, particularly where glucose levels are changing rapidly (Figure 4). Current and recent glucose levels, trend information, and a visual alarm are all presented so that a patient can predict future low or high glucose excursions. Using this information will allow the patient to take actions to spend more time in the euglycemic range and less time in the hypoglycemic or hyperglycemic ranges. This potential decrease in glycemic variability will not necessarily be reflected in an improved A1C value, which reflects mean glycemic levels.
Figure 4.
Tradeoffs between emphasis on high sensitivity compared to emphasis on high specificity in a hypoglycemic alarm that is part of a continuous glucose monitor.
Evidence- Type 1 Diabetes
Studies may be divided according to background therapies (insulin pump or injection therapy).
STUDIES UTILIZING EITHER INSULIN PUMP OR INJECTIONS AS BACKGROUND THERAPIES
-
The seven-country GuardControl Study was the first randomized controlled trial to ever demonstrate a statistically significant improvement in A1C levels with the use of RT-CGM (17). The Guardian RT was used either continuously or biweekly for three months and both regimens were compared to control treatment which did not include use of CGM. At one month and at three months the continuous users had significantly lower A1C levels than the controls. The biweekly users had intermediate improvement which did not reach statistical significance compared to the outcomes in the control group.
-
In 2008, the Juvenile Diabetes Research Foundation Continuous Glucose Monitoring Study Group evaluated 322 adults and children with type 1 diabetes (either injection or insulin pump therapy) and A1C 7-10% who were randomized to either RT-CGM or usual care (18). RT-CGM was associated with a 0.53% reduction in A1C compared to usual care (p<0.001), but was only significant among subjects over age 24 due to lack of consistent use in younger patients. Hypoglycemia was infrequent and was not different between groups.
-
In 2011, 120 children and adults with type 1 diabetes on insulin pump or injection therapy and A1C <7.5% were randomly assigned to RT-CGM (Freestyle Navigator—not available in the US) or masked CGM every other week (19). The time spent in hypoglycemia was reduced over 50% at 26 weeks, and patients spent more time in 70-180 mg/dl range.
-
In the IMPACT trial, 241 adults with type 1 diabetes with an A1C less than or equal to 7.5% were randomly assigned to Freestyle flash glucose monitoring (described in more detail under "Overview of Stand-Alone Personal CGM systems") vs. SMBG. In this group 68% of the patients were treated with multiple daily injections and 32% with CSII. The amount of time spent in hypoglycemia was decreased by nearly 90 minutes per day (P<0.0001) when patients had access to CGM data (20). It must be noted that this technology does not provide real-time alerts for impending hypoglycemia or hyperglycemia and data are accessed via a hand-held device on demand. In a small study of patients with hypoglycemia unawareness or recent severe hypoglycemia, RT-CGM more effectively reduced the time spent in hypoglycemia compared to flash glucose monitoring (21).
STUDIES UTILIZING INSULIN PUMP THERAPY AS BACKGROUND
-
In the largest study to date, the STAR3 study, 485 adults and children with A1C 7.4-9.5% were randomized to sensor-augmented pump therapy (Medtronic Paradigm Revel) or multiple daily injections per day (22). Sensor-augmented pump therapy resulted in better A1C reduction with between-group difference of 0.6%, p<0.001. Hypoglycemia did not differ between groups, but only short-term CGM data were available for comparison and patients with a history of severe hypoglycemia were excluded.
STUDIES UTILIZING INJECTION THERAPY AS BACKGROUND
-
In 2016, a 6-month randomized controlled trial, the DIAMOND study, compared RT-CGM (using Dexcom G4 system) versus SMBG in 158 patients with type 1 diabetes on multi-dose injection therapy and demonstrated a significantly lower A1C (between group difference 0.6%, p< 0.0001), decrease in hypoglycemia (43 minutes vs. 80 minutes per day, p=0.0002) and less glucose variability with RT-CGM compared to SMBG. This study did not address hypoglycemia frequency in the two groups (23).
-
The GOLD trial studied 161 patients with type 1 diabetes receiving multiple daily injections with either RT-CGM (Dexcom G4) or standard care in a random order cross-over trial. The mean difference in A1C was 0.43% (p<0.001), favoring RT-CGM. One subject in the CGM group compared to 5 subjects in the standard care group experienced a severe hypoglycemic event. The percentage of time spent in hypoglycemia numerically favored the CGM group but statistical analyses were not presented. There was a significant reduction in standard deviation and MAGE (measures of glucose variability). Overall well-being, diabetes treatment satisfaction, and fear of hypoglycemia improved (24).
PATIENTS WITH HYPOGLYCEMIC UNAWARENESS
Many older studies specifically excluded patients with a history of severe hypoglycemia or were underpowered to detect significant hypoglycemia. Two recent studies have examined the use of RT-CGM in patients with hypoglycemia unawareness, which is a risk factor for severe hypoglycemia (events requiring outside assistance to treat).
-
In the HypoCOMPaSS trial, 96 patients with a history of hypoglycemia unawareness determined by the GOLD Score of at least 4 or more were randomly assigned in a 2x2 factorial design to insulin pump or injection therapy, both with access to a bolus insulin calculator, and either RT-CGM (Medtronic Continuous Glucose Monitoring System) or SMBG. All patients had diabetes education with a goal toward hypoglycemia avoidance (29). The results demonstrated a similar reduction in severe hypoglycemia and improvement in hypoglycemia unawareness and fear of hypoglycemia without a significant treatment interaction between insulin or glucose monitoring interventions. Treatment satisfaction was higher with insulin pump compared to injection therapy but similar between RT-CGM and SMBG.
-
The IN CONTROL trial evaluated patients with Type 1 diabetes and hypoglycemia unawareness receiving either injection or insulin pump therapy in a crossover study comparing RT-CTM (Medtronic Paradigm Veo system with a MiniLink transmitter and an Enlite glucose sensor) or SMBG (30). Hypoglycemia was significantly reduced with RT-CGM compared to SMBG (including a 9.8% reduction in events <70 mg/dl and 44% reduction in events <40 mg/dl). Severe hypoglycemic events were significantly reduced but hypoglycemia unawareness was unchanged.
Differences between studies may be explained by differences in populations and the technologies utilized. In the In CONTROL study, contact with patients was less frequent, sensor use was greater (89 vs. 57% in HypoCOMPaSS) and there were no insulin adjustment protocols. Therefore, more studies are needed to understand the potential role of background therapy, other technologies, and clinical support.
PATIENT REPORTED OUTCOMES
Generic Quality of life scores generally do not improve with RT-CGM but treatment-specific measures, such as diabetes distress, hypoglycemic confidence, fear of hypoglycemia and to a lesser extent, measures of convenience, efficacy and performance, may be improved (22,31,32).
Evidence- Type 2 Diabetes
In patients with type 2 diabetes, even in patients not on insulin, RT-CGM may act as a motivator and positive influence for patients to improve lifestyle. The change in behavior can potentially lead to better glycemic control (33).
-
In 2012, Vigersky et al. randomized 100 patients with type 2 diabetes on basal insulin and anti-hyperglycemic agents into either a group that used real-time RT-CGM intermittently (2 weeks on, 1 week off) or a group that recorded SMBG four times per day for 12 weeks. At 12 weeks, they found a statistically significantly greater reduction in A1c by 1.0% in the CGM group compared to 0.5% reduction in the SMBG group. The effect persisted up to the 40-week follow-up, 0.8% and 0.5% reduction in A1c in the RT-CGM versus SMBG group respectively (34).
-
In 2017, Beck et al conducted a randomized study to evaluate benefit of RT-CGM use in 158 patients with type 2 diabetes with mean A1C of 8.5% treated using multiple daily injections (35). Over a 24-week period the A1C decreased to 7.7% in the RT-CGM group compared to 8% in the group with usual care (mean difference -0.3%, p=0.022). RT-CGM derived hypoglycemia and quality of life did not differ.
Recommendations
Patients should be adequately informed of the benefits and limitations of this technology, particularly with respect to the role for SMBG. At a minimum, structured education programs encompassing concepts such as carbohydrate counting and active insulin time (insulin on board) should be completed prior to considering RT-CGM, and payers may require that patients demonstrate that they can reliably and consistently perform SMBG (36). Several expert groups have issued guidance in the use of RT-CGM.
-
In 2016, the Endocrine Society, co-sponsored by The American Association for Clinical Chemistry, the American Association of Diabetes Educators, and the European Society of Endocrinology, published guidelines for use of insulin pumps and CGM. The guidelines recommended RT-CGM in adults with type 1 diabetes and any A1C who are willing and able to use the devices nearly daily. The panel suggested short-term intermittent use for patients with type 2 diabetes (not requiring prandial insulin) who had an A1C ≥7% and are willing and able to use the device (4).
-
The American Diabetes Association (ADA) Standards of Care recommend CGM in adults with type 1 diabetes and those with hypoglycemia unawareness or frequent hypoglycemia (Table 2). The guidelines specifically recommend that patients who are older than age 65 continue to have access to CGM. Among pediatric patients, the ADA notes that CGM may reduce missed school days with regular usage (1).
Table 2.
ADA 2020 Recommendations for CGM
View in own window
Real-time CGM in conjunction with insulin therapy are a useful tool to lower A1C levels and/or reduce hypoglycemia in adults with type 1 diabetes who are not meeting glycemic targets,
have hypoglycemia unawareness, and/or have episodes of hypoglycemia. (A)Real-time and intermittently scanned CGM in conjunction with insulin therapy are useful tools to lower A1C and/or reduce hypoglycemia in adults with type 2 diabetes who are not meeting
glycemic targets. (B)CGM should be considered in all children and adolescents with type 1 diabetes, whether
using injections or continuous subcutaneous insulin infusion, as an additional tool to help improve glucose control. (B)Real-time CGM may be used effectively to improve A1C levels, time in range, and neonatal
outcomes in pregnant women with type 1 diabetes. (B)-
A= Clear evidence from well-conducted, generalizable randomized controlled trials that are adequately powered; B= Supportive evidence from well-conducted cohort studies
-
-
The 2016 American Association of Clinical Endocrinologists recommendations for use include all adults and children with type 1 diabetes, especially those with severe hypoglycemia or hypoglycemia unawareness, and all patients with type 2 diabetes on multiple insulin injections, basal insulin, or sulfonylureas who are at risk for hypoglycemia (2).
-
In 2017, the Advanced Technologies & Treatments for Diabetes (ATTD) Congress organized an international consensus panel, consisting of physicians, researchers, and individuals with diabetes to analyze the existing literature and to provide guidance for utilizing, interpreting, and reporting CGM data (37). This was updated in 2019 (Table 3, 4). These recommendations are supported by recent data from the DCCT demonstrating that a 10% reduction in time in target glucose range derived from 7-point self-monitored glucose profiles is associated with a 40% reduction in risk of microalbuminuria and a 64% reduction in risk of incident or progressive retinopathy (38).
Table 3.
CGM-Based Targets for Different Diabetes Populations
View in own window
Glucose Range %Time in Range Non-Pregnant Patients Type 1 and Type 2 Diabetes Older/High Risk Diabetes >250 mg/dl (13.9 mmol/L)* <5% <10% <180 mg/dl (10 mmol/L) <25% <50% 70-180 mg/dl (3.9-10 mmol/L) >70% >50% <70 mg/dl (3.9 mmol/L)** <4% <1% <54 mg/dl (3.0 mmol/L) <1% Pregnant Patients Type 1 Diabetes Gestational and Type 2
Diabetes #>140 mg/dl (7.8 mmol/L) <25% - 63-140 mg/dl (3.5-7.8 mmol/L) >70% - <65 mg/dl (3.5 mmol/L) <4% - <54 mg/dl (3.0 mmol/L) <1% - - *
-
Includes time >180 mg/dl, **Includes time <54 mg/dl, #Insufficient data
Table 4.
Summary of ATTD Recommendations for CGM
View in own window
Limitations of A1C CGM should be utilized when there is a discrepancy in A1C and other measures of glucose control
CGM should be utilized to assess hypoglycemia and glucose variabilityGuiding management and assessing outcomes CGM should be considered for patients with type 1 diabetes and insulin treated type 2 diabetes who are not achieving targets or those with hypoglycemia
All patients should receive training education regarding how to interpret and respond to their data, utilizing standardized programs with follow-upPerformance No accepted standard exists for CGM system performance. However,
a mean absolute relative difference ≤10% provides little additional
benefit for insulin dosing.Definition and assessment of hypoglycemia Clinical classification
Level 1: 54-70 mg/dl with or without symptoms
Level 2: <54 mg/dl with or without symptoms (clinically significant)
Level 3: cognitive impairment requiring external assistance for recovery
Quantification using CGM
% of values or time below a given threshold (54 or 70 mg/dl) Number of events (defined as CGM readings persistently below threshold for at least 15 min. with recovery defined as persistent readings over the threshold for at least 15 min.) over a given reporting period)Glycemic Variability Coefficient of Variation should be the primary measure Time in Range The % time in hyperglycemia, hypoglycemia, and target range should
be reported.CGM Metrics Standardized reporting using the AGP and integration into electronic health records is recommended.
Key metrics:
--Number of days worn (14 days recommended)
--% time CGM is active (70% of data from 14 days recommended)
--Mean glucose
--Glucose Management Indicator
--Glycemic variability (%CV, target <36%)
--% Time in Range (TIR): 70-180 mg/dl (3.9-10.0 mmol/L)
T%--Time Above Range (TAR): 181-250 mg/dl (10.1-13.9 mmol/L), and >250 mg/dl (>13.9 mmol/L)
--%Time Below Range (TBR): 54-69 mg/dl (3.0-3.8 mmol/L), and
<54 mg/dl (3.0 mmol/L) -
Also, in 2017 the ADA and the European Association for the Study of Diabetes published a joint statement providing recommendations for systematic improvements in clinical use and regulatory handling of CGM devices (39).
-
In 2016, the Diabetes Technology Society sponsored a panel of experts in inpatient diabetes management to review the evidence for us of CGM in the hospital (40). The panel agreed that CGM had the potential to improve clinical outcomes, particularly for patients who are unable to communicate signs or symptoms of hypoglycemia, but use is limited by lack of data demonstrating accuracy (particularly in the hypoglycemic range or in case of diabetic ketoacidosis, poor perfusion, or acetaminophen use) and clinical utility, and a lack of decision support systems, including infrastructure for communicating results to care teams and to the electronic medical record. The panel agreed that patients who are admitted with personal CGM devices should be allowed to continue use of such devices under the condition that they are able to self-manage the devices on their own and are followed by an endocrinologist or experienced practitioner who is specifically trained in their use. In particular, the panel advised implementing institutional policies that recommend continued capillary or blood glucose monitoring, ensuring that CGM data are not used for inpatient insulin dosing (since no CGM device is FDA approved in the inpatient setting), and requiring patients to sign safety waivers which illustrate the potential risks and benefits of continued use. Devices must be removed for any MR or CT imaging.
Limitations of Use
It should be emphasized that most prospective randomized controlled trials enroll highly motivated patients. In the case of the STAR3 trial, access to a computer was required. In the real-world setting, there are concerns about limited resources for training, and less motivated patients may be overwhelmed with the additional data, particularly where complex algorithms are required. Nevertheless, in the Type 1 Diabetes Exchange Registry, CGM use increased from 7% in 2010-2012 to 30% in 2016-2018, and rose more than 10-fold in children (41).
HbA1c levels were lower in CGM users compared to nonusers. While CGM use has improved substantially over time, more than half of respondents cited cost or insurance coverage as a significant barrier to use (42). Modifiable reasons for avoiding use include the hassle of devices (47%) and aversion to having a device attached to the body (35%). In a multi-national study of 263 patients, persistent sensor use for 12 months was only 30% (43). Improvement in A1C was associated with higher A1C at baseline, older age, and more frequent sensor use. Diabetes related hospital admissions were reduced following the initiation of sensor augmented pump therapy and fear of hypoglycemia improved. In the 6-month follow-up phase of the JDRF-CGM trial, RT-CGM was initiated in the control group in a manner that more closely approximates clinical practice (44). Investigators found a significant reduction in CGM use in all age groups over time. However, increasing sensor use was associated with A1C reduction. Moreover, in a study of over 29,000 pediatric patients with type 1 diabetes in the Type 1 diabetes Exchange Registry or the German/Austrian DPV Initiative, pediatric CGM use was associated with lower mean HbA1c regardless of insulin delivery modality (pump or injection (45). It is likely that adherence will improve as technologies improve.
Other limitations include possible interference with acetaminophen, ascorbate, and other active agents in glucose-oxidase based electrochemical sensors. They are also dependent on both the sensitivity and specificity on the enzyme availability on the electrode surface. There are well known delay artifacts due to the time lag between glucose concentration in the interstitial fluid and blood glucose. These time ranges, often between 5 and 10 minutes, are not crucial to analyzing retrospective data, but can be critical when CGM is indicated for real-time decision making (46).
Daily Use
Patients must be aware that sensor readings can deviate from actual blood glucose measurements, particularly during rapid glucose changes such as that which occurs post-meal or during exercise. Calibration, where necessary, should not be performed when trend arrows indicate rapid swings in glucose. While systems are becoming more reliable, patients may need to verify sensor readings before taking action such as meal boluses or treatment of hypoglycemia depending on the device, even if a device is approved for nonadjunctive use.
Alarm thresholds should be set in order to maximize patient compliance, keeping in mind that the sensitivity for detecting hypoglycemia decreases as the threshold is reduced below 70 mg/dl (47). Conversely, specificity improves to a much smaller degree at lower thresholds, and thus false alarms may not be reduced substantially.
Several algorithms have been published that provide specific guidance to patients for responding to trend arrows and alarms and are summarized below. All algorithms are complex and are not integrated within bolus calculators of existing insulin pumps. Therefore, they should only be implemented in patients who have demonstrated an understanding of CGM technology, including lag times between CGM and BGM, calibration procedures, alerts and trend arrows, as well as understanding of insulin action time and the risks of insulin stacking. In one small study, trend arrows were accurate approximately 79% of the time outside of mealtime windows (30 minutes before and 120 min after carbohydrate intake) but this dropped to ~60% within mealtime windows (48). Thus, algorithms are not intended for use post-meal. The use of automated insulin delivery systems should increase safety and efficacy and reduce the complexity of the trend arrow approach.
-
The algorithm by Jenkins et al. provides tiered recommendations that are based upon the meter glucose and sensor trend arrows (49). In addition, the algorithm advises patients how to review downloads of the data periodically (weekly) and make adjustments. Patients who were randomly assigned to sensor augmented pump with the algorithm had lower A1C and reported better quality of life at 16 weeks compared to patients who did not get the algorithm. The effect on quality of life persisted at the 32-week follow-up, and was associated with A1C reduction. Importantly, patients who received the algorithm at 16 weeks after initiating sensor augmented pump did not benefit.
-
The DirecNet study algorithm (for use with the Navigator system) recommended that patients increase or decrease the meal + correction bolus by 10-20% based upon the rate of change and provided specific instructions for responding to alarms (50). Algorithm use was high in the first 3 weeks but dropped off by week 13, despite increasing insulin self- adjustments, possibly as patients became more independent over time.
-
Subsequent methods recommended adjustment of only the correction insulin dose by the amount needed to cover a glucose level that is incrementally higher or lower than the current glucose, based upon the trend arrow (51,52).
-
Klonoff and Kerr proposed a more straightforward correction dose (in 0.5-unit increments), based upon the trend arrow and the patient's insulin sensitivity (53).
-
A consensus statement facilitated by the Endocrine Society provides expert guidance on the use of trend arrows for making treatment decisions (54). The guidance recommends adjustment of boluses pre-meal and no sooner than 4 hours post-meal in 0.5-unit increments based upon the trend arrow and the patient's sensitivity. The statement recommends no additional treatment within 2 hours of a previous meal bolus, and correction bolus using the bolus calculator or usual correction dose only in the 4 hours after a meal. Similar expert guidance has been developed for the Freestyle Libre system (55).
Overview of Stand-alone Personal CGM and Flash Glucose Monitoring Systems
The first RT-CGM (Guardian, MedtronicR) was approved in 2004. Since then, additional models and other devices have entered the market, and accuracy and patient satisfaction have improved. Several personal continuous glucose monitors have been approved by the US Food and Drug Administration (FDA) for use in the United States or carry CE marking for use in Europe and are currently on the market (Table 5). For a full review of regulatory requirements for glucose monitoring devices, the reader is referred to one of several excellent reviews (56).
Table 5.
Comparison of Subcutaneous Continuous Glucose Monitoring Devices
View in own window
Calibration required | Confirmatory Fingersticks required prior to treatment | Real-time alerts | Sensor Life (days) | Warm-up (hrs) | Remove for MRI, CT diathermy | Acetaminophen interference | |
---|---|---|---|---|---|---|---|
Dexcom G5 | Y | N | Y | 7 | 2 | Y | Y |
Dexcom G6 | N | N | Y | 10 | 2 | Y | N |
Medtronic Guardian 3 | Y | Y | Y | 7 | 2 | Y | Y |
FreeStyle Libre | N | N | N | 14 | 1 | Y | N |
Freetyle Libre | N | N | Y | 14 | 1 | Y | N |
Eversense (surgical implant) | Y | N | Y | 90* | NA | Y | No |
- *
-
180 days approved in Europe
GUARDIAN RT
The Guardian RT was approved in 2005 for patients over 18 years of age. The sensor and monitor are connected through a wireless transmitter and the monitor displays real-time glucose results every five minutes. The continuous data can be stored up to 21 days and downloaded any time into a computer. The data can then be reviewed with proprietary software provided by the manufacturer. The original Guardian and Guardian RT devices contained a large transmitter piece and were used primarily for research. A smaller data transmission system, known as the Minilink, to send glucose information from the sensor to the belt-attached monitor, was approved in 2007. This component was immediately incorporated into the Guardian RT. Sensors are FDA approved for 72 hours of use. The monitor is similar in appearance to the Paradigm Revel sensor augmented pump (discussed below). However, it functions only as a CGM and not as a pump.
GUARDIAN CONNECT
The Guardian Connect utilizes the Medtronic Guardian Sensor 3, the Guardian Connect transmitter, and the Guardian Connect app to transmit data via Bluetooth every 5 minutes to the user's smart phone or device (initially only available on iOS devices) via the Guardian Connect App or Sugar.IQ app on smartphones and via CareLink personal and professional software. A separate receiver is not available with this system. Data can be shared with others remotely, and SMS messages can be sent in times of hypoglycemia. The system is only approved for adjunctive use and at least 2 daily fingerstick calibrations are required.
DEXCOM G4 PLATINUM
The Dexcom CGM utilizes a glucose oxidase sensor at the tip of a wire that is implanted in the subcutaneous space (Figure 5). The data are transmitted wirelessly and are displayed on a separate receiver. This device is FDA approved to provide glucose readings for 168 hours or 7 days. The device must be calibrated every 12 hours. Real-time data may be shared with up to 5 other individuals using the Dexcom Share app. The G4 works with a Windows based software called DexCom Studio or Dexcom Clarity Web-based data management software for retrospective downloading and analysis of glucose data. The G4 is compatible with older models of insulin pumps (Animas Vibe and the T-slim G4) but consists only of the integration of glucose values and alerts within the pump display and glucose values do not affect insulin delivery. Dexcom devices can also be operated in blinded mode for professional CGM applications (discussed above). In the REPLACE-BG Trial, patients with type 1 diabetes were randomly assigned to use of Dexcom G4 with (N=77) or without (N=148) confirmatory blood glucose monitoring (57). Patients in the CGM only group used blood glucose monitoring only for calibration, sick days, acetaminophen use, bolusing if the glucose was >250 mg/dl or fasting or persistent glucose >300 mg/dl. After 26 weeks, the % time in target range 70-180 mg/dl was 63% in the CGM only group and 65% in the CGM plus blood glucose monitoring. There was no difference in hypoglycemia, hyperglycemia, or A1C. There were no cases of diabetic ketoacidosis or severe hypoglycemia, though patients at high risk for these events were excluded from participation in the study.
Figure 5.
Dexcom G4 Platinum (clockwise) applicator containing a sensor ready for insertion, transmitter which will be attached to the sensor after insertion, and receiver.
DEXCOM G5 MOBILE
The Dexcom G5 mobile provides increasing sensor accuracy and allows users to obtain glucose monitoring data and alerts directly via their smart device and share data with others via the Dexcom Share App (figure 6). A fingerstick confirmation is not needed for making treatment decisions, except in situations in which the glucose is rapidly changing, or glucose value is unexpected and does not match the patient's symptoms. Like the G4, calibration is required every 12 hours and the device is inaccurate in the setting of acetaminophen use.
Figure 6.
Dexcom G5 System (transmitter shown with receiver. Data and alerts may alternatively be accessed using a smart device)
DEXCOM G6 MOBILE
The Dexcom G6 is similar to the G5 but has a longer sensor life of 10 days, no longer requires calibrations, and minimizes interference by acetaminophen. G6 is also associated with a smartphone app that allows the patient to log activity, set reminders or alarms, and physically see their glucose levels and trends throughout their time wearing the device. The Dexcom CLARITY Diabetes Management Software organizes and presents the patient's blood glucose data.
DEXCOM G7 MOBILE
The Dexcom G7 is tentatively scheduled to be released in late 2020 and will feature a thinner, less expensive, fully disposable device that may be worn for ~14 days.
FREESTYLE LIBRE
The sensor utilizes Wired Enzyme™ technology in which the enzyme and mediator are co- immobilized on the sensor (figure 7). It offers factory calibration, and therefore nearly eliminates the need for fingerstick monitoring. However, patients are still advised to perform SMBG whenever an alert appears on the reader display (which occurs when the glucose is rising or falling rapidly) or whenever the glucose value does not fit the patient's symptoms. The reader contains a built-in meter for this purpose. The sensor is FDA approved for 14 days of use. The system is not approved for use in children under age 18, or during pregnancy or in persons requiring hemodialysis. The Libre has minimized the interference by acetaminophen which is present in other devices but interference from other substances such as ascorbic acid or aspirin may be possible. The Libre also differs from other CGM devices in that the system does not alert the user for glucose values surpassing a high or low threshold. In addition, glucose values are not automatically made available to the user but are easily and instantly accessed by scanning the sensor with a handheld reader or the associated app FreeStyle LibreLink.
However, this product may be attractive option for patients who are averse to the hassle imposed by other RT-CGM devices. Glucoses are measured every minute and recorded every 15 minutes. Data can be accessed using the reader or downloaded to LibreView cloud based online management system, or using the FreeStyle Libre desktop software. The MARD is reported by the manufacturer to be 9.7% overall, and as with other CGM devices, less accurate on day 1 of wear and in hypoglycemia range (58).
Figure 7.
FreeStyle Libre continuous glucose monitor.
FREESTYLE LIBRE 2
The FreeStyle Libre 2 system offers real-time alerts for high or low glucose values and improved accuracy (59).
EVERSENSE
The Eversense system (Senseonics, Figure 8) is a 90-day implantable sensor that uses fluorescent technology to send measures via a rechargeable transmitter which rests just above the skin to a smartphone app titled Eversense NOW (60). In a pivotal clinical study of 71 patients with type 1 and type 2 diabetes, there were no device-related serious adverse events, and the MARD was 11.1%, with over 99% of samples in clinically acceptable error zones A and B of Clarke Error Grid Analysis (61). One study reported interference with tetracycline and mannitol, but not with acetaminophen or ascorbic acid (62). The Eversense XL CGM system consists of a 180-day implantable sensor that has been shown to have acceptable safety and accuracy and is currently approved for use in Europe (63). This option may be particularly useful for patients with privacy concerns, physical disability, needle phobia, allergies or other difficulty with adhesion, or activities or professions that may be barriers to external wear (64).
Figure 8.
Eversense XL Implantable CGM.
Sensor Augmented Pumps
To date the largest A1C reductions have been observed when sensors are initiated with insulin pump technology. In the observational (nonrandomized) COMISAIR study, patients initiating CGM (with or without insulin pump) achieved significantly larger reductions in A1C (-1.2%) compared to subjects initiating insulin pump alone (-0.6%) or remaining on injections alone (- 0.3%) (65). There was no difference in outcomes between the DexCom G4 and Enlite sensor.
A reduction in time spent in hypoglycemia was observed only in patients using CGM (8% vs 6%, p<0.001).
Currently, several sensor augmented insulin pump systems are available. The Minimed Paradigm REAL-time Revel sensor-augmented pump system (Medtronic Diabetes, Northridge, CA) utilizing the Guardian RT CGM was the first such system to become available (Figure 9). The other Medtronic systems with closed loop technologies are discussed in subsequent sections.
Other sensor and pump manufacturers have been in discussions or held agreements to develop and market sensor augmented pumps. The Animas Vibe featured integrated Dexcom G4 Platinum CGM but was discontinued by the manufacturer in 2017. Another system, the Tandem t:slim G4 insulin pump provides integrated Dexcom G4 data on the pump display but does not interact with insulin administration. The t:slim X2 was FDA approved in 2017 and incorporated the Dexcom G5 CGM. The newest t:slim utilizes Dexcom G6 for automated insulin delivery (discussed below).
Figure 9.
The Paradigm sensor augmented pump consisting of a sensor (A), which is attached to a Minilink transmitter (B) and communicating with an insulin infusion pump (C). Screen displays information about: (D) An alarm warning; (E) A trend graph portraying recent patterns of glycemia, (F) Glucose level, (G) Trend arrow for the direction and magnitude of trends in glycemia.
STEPS TOWARDS AN ARTIFICIAL PANCREAS
Until recently, RT-CGM technology has operated completely independently of insulin delivery. By combining continuous basal insulin delivery during fasting periods with discrete bolus doses of insulin at mealtimes, insulin delivery can be crafted to mimic the natural pattern of pancreatic insulin release. An artificial pancreas consists of: 1) an automatic and continuous glucose monitor; 2) an implanted continuous insulin delivery system; 3) a control processor to link the insulin delivery rate to the glucose level; and 4) a signal to send the glucose level to the body surface for continuous display onto a monitor. Limitations to full implementation include sensor accuracy and lag time, inadequate onset and offset of currently available rapid acting insulin analogs, meal challenges, and changes in insulin sensitivity due to circadian rhythms, exercise, menstrual cycles, and intercurrent illness (66). However, even incremental advances are likely to expand access of this technology to more patients since they may improve glucose control without increasing the complexity of decision-making on the part of the patient. The long-term safety, efficacy, cost, and cost-effectiveness of an artificial pancreas are still largely unknown at this time. However, the urgency of this technology is demonstrated by the #WeAreNotWaiting movement, which has given rise to home-grown, crowd-sourced, patient driven systems that utilize existing devices which are linked by open-source software, such as Open Artificial Pancreas System, and Loop (for a recent blog entry, see http://www.diabettech.com/looping-a- guide/). A 2019 retrospective observational study of patients with Type 1 diabetes demonstrated lower mean glucose, higher time in target range, and less time in hypoglycemia using Open Artificial Pancreas Systems (OpenAPS) compared to sensor augmented pump use alone (67). None of these systems are FDA approved though, they are being more systematically studied (68). Therefore, they may carry safety concerns, particularly among less tech-savvy patients. The FDA recently released a warning on the risks associated with unapproved or unintended use of diabetes devices (69). The reader is referred to one of several recent reviews as a detailed review is beyond the scope of this chapter (70,71).
Threshold Suspend
Progress is expected toward a fully functional closed loop system in incremental steps. The first step toward a fully automated "artificial pancreas" is the low glucose suspend feature, which is now available. The Medtronic 530G system, containing the Veo insulin pump and Enlite sensor, is the first sensor augmented pump with low threshold suspend and uses the same sensor as the more recent 630G system. The Enlite sensor accuracy is significantly improved over the previous Sof-sensorR, with an MARD of 13.6% when used with the 530G (72). The Enlite is also one-third of the size of Sof-sensor and the filament is 38% shorter. The Enlite sensor may be worn up to six days. The low threshold suspend SmartGardTM technology suspends the pump for up to two hours in the event of sensor detected hypoglycemia in which the user does not respond to the alarm. Prior to suspension, a "siren" sounds which is distinct from other high or low alerts, and the suspension can be overridden at any time. The MiniMed Connect mobile accessory sends sensor data to an app on a mobile device where data can be viewed (available only with the 530G system). A study that enrolled 247 patients with type 1 diabetes and documented nocturnal hypoglycemia to sensor-augmented pump with or without a low-glucose threshold-suspend feature demonstrated similar A1C between groups at 3 months but lower frequency of nocturnal hypoglycemia (73). Similar findings were demonstrated in an Australian study of 95 patients, in which the incidence rate ratio for hypoglycemia was 3.6 (95% CI 1.7-7.5, p<0.001) (74). There were no reports of DKA in either study.
Suspend Before Low
The next incremental step in closed loop systems is the suspend before low feature, currently available in the Medtronic 640G (approved only in Europe) and the 670G systems. This feature automatically suspends insulin delivery 30 minutes before a low glucose threshold is predicted and resumes delivery once the glucose recovers, without alerting the patient. In a 6-month randomized study of 154 children and adolescents with type 1 diabetes, the 640G system reduced the time spent in hypoglycemia from 2.6 to 1.5% without causing a change in HbA1c (75). The t:slim X2 Insulin Pump incorporates Basal IQ technology with predictive low glucose suspend using the Dexcom G5 or G6 sensor. In a randomized cross-over study of 103 participants with type 1 diabetes age 6-72 years of age, predictive low glucose suspend resulted in a 31% reduction in time spent in hypoglycemia < 70 mg/dl without a change in mean glucose or time in hyperglycemia (76).
Hybrid Closed Loop (HCL)
This step refers to sensor glucose driven automatic adjustment of basal insulin, which still requires the patient to bolus for meals.
MEDTRONIC 670G
The first system to gain FDA approval is the Medtronic 670G (Figure 10), which adjusts basal insulin delivery every 5 minutes when in auto mode. This system utilizes the Guardian sensor 3, which offers enhanced sensor accuracy, with an overall MARD of 9.64% (77). The system was associated with a reduction in A1C from 7.4 to 6.9% and there were trends in improvement of time in target range and hypoglycemia in a non-randomized study of 124 patients with type 1 diabetes (78). There are few studies addressing long-term use however. In a 1-year prospective observational study of 84 patients, 28% stopped using auto mode by 3 months, and 33% discontinued by 12 months (79). The most common reasons for discontinuation included sensor issues (62%) and difficulty obtaining supplies (12%).
Figure 10.
The 670G Hybrid Closed Loop insulin delivery system consisting of the Guardian sensor 3 (A), which is attached to a Guardian Link 3 transmitter (B) and communicating with an insulin infusion pump (C).
TANDOM CONTROL-IQ
This system utilizes a t:slim X2 insulin pump with a calibration-free Dexcom G6 sensor (81). In a 6 month trial of 168 patients (age 14-71) randomized 2:1 to hybrid closed loop vs. sensor augmented pump alone, the % time in target 70-180 mg/dl was increased by 11% more in the hybrid closed loop group compared to sensor augmented pump (p<0.0001), with improvements in hypoglycemia, mean glucose and A1c.
SMART PHONE ALGORITHM
A 12-week study randomly assigned 86 participants with Type 1 Diabetes aged 6 years and older treated with insulin pump and with suboptimal glycemic control to receive either hybrid closed-loop therapy (using a Model predictive control algorithm on a smart phone, not FDA approved) or sensor-augmented pump therapy. The study found that hybrid closed-loop insulin delivery improves glucose control while reducing the risk of hypoglycemia across a wide age range in patients with suboptimal control of Type 1 Diabetes (82).
OMNIPOD HORIZON
This is a HCL system currently under development using the Omnipod DASH platform (83).
Closed Loop Systems (CLC)
Additional steps toward closed loop control (CLC) insulin delivery require algorithmic insulin adjustments, which arguably present additional safety concerns. Overnight CLC insulin delivery is relatively straightforward, whereas post-meal control and exercise effects remain the most challenging of events to manage. Thus far, most randomized studies have been small and reported only short-term outcomes, often in controlled settings. Systems have utilized single hormone (rapid acting insulin only) or dual hormone (both fast-acting insulin analog and glucagon to imitate normal physiology) as directed by a computer algorithm (Figure 11) (84). At this time, there are insufficient data demonstrating the superiority of one system or algorithm compared to others. A meta-analysis of 40 randomized studies (35 studies using insulin alone and 9 dual hormone studies) including 1027 participants with type 1 diabetes demonstrated a significant increase in % time in target range (70-180 mg/dl, weighted mean difference 9.6%, 95% CI 7.5-12%), as well as less time in hypoglycemia or hyperglycemia, regardless of type of system (85). In another meta-analysis of 24 studies and 585 participants (7 studies using dual- hormone therapy and 20 studies of insulin only) reported greater improvement in time in target with artificial pancreas systems (12.6%, 95% CI 9.0-16.2, p<0.0001), and greater improvement with dual hormone compared to single hormone systems (86). A brief overview of recent studies is presented below.
Figure 11.
Dual hormone Closed Loop Control System.
SINGLE HORMONE CLC SYSTEMS
In one of the first randomized studies, 56 children and adolescents attending diabetes camps received sensor augmented pump or artificial pancreas (via fuzzy logic controller) on two separate nights in random order (87). The artificial pancreas demonstrated markedly reduced hypoglycemic events (7 vs. 22 events in the artificial pancreas compared to the sensor augmented pump, p=0.003) and time in hypoglycemia, as well as better overall mean glucose and glycemic variability.
In another study, 32 adolescents with type 1 diabetes attending a 5-day ski camp were randomized to CLC or remotely monitored sensor-augmented pump. In the CLC group time in range increased by 7% (1 hour and 40 minutes per day) when compared to the sensor- augmented pump group. At the beginner ski level, percent time spent in blood glucose <70mg/dl decreased by almost 50% but this was not observed in the advanced ski level, possibly due to more experience with prevention and management of hypoglycemia (88).
In a cross-over study of 40 patients with type 1 diabetes, there was a statistically significant increase in percentage of time in range with CLC when compared to sensor augmented pump over 24 hours, 78.3% vs 71.4% respectively during the day and 85.7% vs 67.6% overnight. The CLC group spent a lower percentage of time in low glucose range of less than 70mg/dl when compared to the sensor augmented pump group, 2.5% vs 4.3% respectively with p-value=0.002 (89).
DUAL HORMONAL CONTROL
In 2014, Russell et al conducted a random order cross-over study to evaluate glycemic control with a dual hormone (glucagon and insulin) CLC system compared to CSII in 32 adolescents and 20 adults with type 1 diabetes in a real-world outpatient setting. Over 5 days of use both groups had a statistically significant reduction in mean sensor glucose from 158 mg/dl to 142 mg/dl and from 159 mg/dl to 133 mg/dl in adolescents and adults respectively. The percentage of time spent in glucose <70mg/dl was lower and statistically significant in the adults (7.3 vs.
4.1%, p=0.01), but did not reach statistical significance in adolescents with dual hormone CLC (4.9 vs. 3.1%, p=0.05) (90).
The same group further evaluated whether an adaptive meal-priming insulin bolus that automatically adjusts the size of breakfast, lunch, and dinner doses by delivering 75% of the average prandial insulin provided for previous meals at that time of day improved glucose control compared to no meal announcement during dual hormone CLC system. Among 12 adults and 12 adolescents with type 1 diabetes, using only weight as the initial input, adaptive meal time boluses resulted in improved mean glucose without increasing time spent with glucose <60mg/dl (91).
In 2016, Haidar et al performed a 3-arm random order crossover study among 21 adults and 7 adolescents with type 1 diabetes comparing dual hormone CLC, single hormone CLC, or insulin pump on overnight glucose control following a high fat, high carb meal or exercise the previous evening (92). The percentage of time spent in target range (70-180 mg/dl) was 81, 76, and 47% in the dual hormone, single hormone, and insulin pump respectively (p<0.001 for each CLC system vs. sensor augmented pump alone). The percentage of time below 72 mg/dl was 5, 1, and 14% in the respective groups and was significantly lower for both CLC groups when compared to conventional therapy (93). However, in a subsequent study, the dual hormone system resulted in significantly less hypoglycemia than a single hormone system (94).
In 2017 this group further evaluated the impact of dual-hormone artificial pancreas on risk of hypoglycemia when compared to single hormone artificial pancreas in 23 adult patients with type 1 diabetes in an outpatient real world setting for 60 hours (95). They noted a median difference of -2.3% between the dual and single hormone system for time spent below 4 mmol/L (72mg/dl) and a median difference of -1.3% between the two groups for time spent below blood glucose of 3.5 mmol/L (63mg/dl). Although they noted numerically less risk of hypoglycemia with the dual hormone system, the results were not statistically significant. Both systems were superior to sensor augmented pump therapy in terms of percentage of time spent in hypoglycemia.
In 2018, a randomized cross-over study compared glucose control over a single night with dual- and single-hormone artificial pancreas systems in subjects with type 1 diabetes and hypoglycemia unawareness (N=18) or hypoglycemia awareness (N=17). This study found that both systems actually performed equally well in terms of preventing nocturnal hypoglycemia in participants with or without hypoglycemia unawareness (96).
Russell and colleagues performed an 11-day random-order cross-over study among 43 subjects with type 1 diabetes under free living conditions. The mean glucose was 140 vs. 162 mg/dl during dual hormone CLC vs. control periods (p<0.0001) and the time spent <60 mg/dl was reduced (0·6% versus 1·9%, p<0.0001) (97).
MINIMALLY INVASIVE AND NONINVASIVE GLUCOSE MONITORS
Continuous hypoglycemia detection systems using current sensing technology must be either implanted (fully or partially, either subcutaneously or into a blood vessel). Implantation is more secure, but may be associated with biocompatibility problems or local irritation. Less invasive methods may be categorized as minimally invasive or noninvasive. Minimally invasive techniques extract fluid (tears or interstitial fluid) while noninvasive technologies do not.
Minimally invasive methods include electrical, nanotechnology, and optical approaches while noninvasive techniques rely on some form of radiation without the need to access bodily fluids. Noninvasive methods frequently incorporate electric, thermal, optical, or nanotechnology methods for detection. Many noninvasive devices under development are aimed for non- continuous monitoring as they often require controlled surroundings including factors such as light, motion and temperature.
-
Optical approaches utilize reflective, absorptive, or refractive properties of infrared and optical bands of the light spectrum to detect glucose. Pure optical methods under development utilize Raman and Near infra-red spectroscopy.
-
Thermal methods detect glucose via the far-infrared band of the spectrum and provide noninvasive approaches for glucose monitoring.
-
Electric methods use electromagnetic radiation, currents, or ultrasound approaches to detect dielectric properties of glucose. Reverse iontophoresis has been employed with early minimally invasive approaches while bioimpedance spectroscopy has been used in recent noninvasive approaches.
-
Nanotechnologies aim to miniaturize existing technologies, including fluorescence and surface plasmon resonance (SPR) approaches (98).
Few devices (other than interstitial CGMs discussed above) have demonstrated high levels of accuracy recommended by expert groups, though several have been approved by CE or FDA (56,99).
Minimally Invasive Glucose Monitoring
REVERSE IONTOPHORESIS
Methods for harvesting interstitial fluid from the body to measure with an external non-implanted sensor are being developed that disrupt the skin barrier and trap the fluid that rises to the surface (reverse iontophoresis).
-
GlucoWatch: The GlucoWatch G2 Biographer (formerly Cygnus, Inc., Redwood City, CA) is one such device but was taken off the market in 2007. In one study, only 20% of participants were still using the device at 18-months (100). The device suffered from inaccuracy, and 49% of wearers reported skin reactions as the reason for discontinuation.
-
SugarBEAT: (Nemaura Medical). This device consists of a daily patch on the abdomen or extremity that passes a small electric current through the skin to draw a measurable amount of interstitial fluid and was CE approved in 2016 for adjunctive use (requires fingerstick confirmation for dosing insulin) (101).
MINIATURE MICRONEEDLES
Several research groups are working with new miniaturization processes specific to microneedles that can access interstitial fluid without any sensation of discomfort or pain. Products such as the K'Watch by French company PKVitality is approved in Europe and are close to commercialization (102).
Noninvasive Glucose Monitoring
Noninvasive glucose monitoring depends either upon on the application of optical, thermal, or electric waves into tissue followed by measurement of the interaction of the energy with glucose in the intravascular, interstitial fluid, and intracellular compartments, or else measurement of a physiologic phenomenon which is proportionate to the blood glucose level. Methods utilizing near infrared, impedance, occlusion, Raman or radio wave spectroscopy on the wrist, finger,
abdomen, or earlobe are completely noninvasive, but suffer from accuracy problems and therefore are thus far only intended for adjunctive use (require fingerstick glucose confirmation prior to dosing insulin). Though several methods have been CE approved, none have been approved by the FDA (71). Devices under development can be classified as taking a measurement either intermittently or continuously.
NEAR INFRARED SPECTROSCOPY (NIR)
Noninvasive testing using infrared light spectroscopy to measure reflection of infrared light from the skin in proportion to the glucose concentration must distinguish the signal of water (which is very large) from that of glucose (which is much smaller), as well as other potential interferents in the skin. However, other analytes that are not a problem for existing invasive monitors can be confounding if their optical properties overlap those of glucose.
-
The Tensor Tip Combo glucometer (CNOGA Medical): This device serves as a traditional glucometer and also noninvasively measures glucose using NIR on the finger. It is currently approved for adjunctive use in Europe and other countries with a MARD of 14% (104).
-
Hello Extense (World Global Network): This device utilizes NIR measurements to estimate a "Sugar Trend" rather than a point of care glucose. The device is approved by the CE and requires calibration with a fingerstick glucose and may not be used for insulin dosing (105).
-
Wizmi (RSP Systems): This system utilizes NIR spectroscopy for continuous glucose monitoring and showed initial MARD of 7.2%. Clinical trials are underway (106).
MID-MILLIMETER WAVE TRANSMISSION SPECTROSCOPY
Mm-Wave transmission spectroscopy is a process by which glucose measurements are obtained from the change in permittivity of radio waves across a medium, which varies with the glucose concentration. GlucoWise (Mediwise) is an example of a device that uses two microstrip patch antennae to measure the change in permittivity of mm (65 Hz) waves (107) and is currently in clinical trials (108).
COMBINATION APPROACHES
Combination approaches take advantage of the collective strengths and weaknesses of multiple individual approaches in order to improve device accuracy.
-
GlucoTrack: The GlucoTrack (Integrity Applications Ltd.) utilizes noninvasive ultrasonic, electromagnetic, and thermal methods to detect glucose-related shifts in earlobe tissue. The device is commercially available and CE approved, but accuracy remains a limiting factor with a MARD of 20% (109).
MOBILE TECHNOLOGY AND DECISION SUPPORT
It has become increasingly clear that the isolated use of glucose monitoring technologies without a plan for using the data provides minimal benefit, particularly among patients with type 2 diabetes or who are not using insulin (110). In order for glucose monitoring to provide the most benefit, patients and providers must be able to easily obtain and communicate the data. Data must be organized in such a way that patterns can be identified, and patients must receive feedback at the point of care. The widespread use of mobile devices provides opportunities for data collection, analysis, and communication of results with health care providers as well as facilitates digital or remote clinical models of care (111). Finally, as healthcare providers are inundated with more data and spend increasing amounts of time using electronic medical records, it has also become paramount that devices and or reports from the devices communicate or interface with these systems (112).
Hurdles to wider implementation of mobile technology include the lack of usability (both for patients, as well as providers who may be expected to review and act upon reports), safety, efficacy (including long-term adherence), and cost-effectiveness studies (113). The lack of data is in part due to the rapidly changing technology itself, which renders the technology obsolete by the time a vigorous clinical trial is conducted and published. The fee for service model is a major barrier to adapting many glucose monitoring technologies, which often require frequent feedback and treatment adjustments, efforts that are not reimbursed without an actual office visit. Finally, cyber security is a big concern for all medical devices, especially for devices that are controlled by a smartphone (114).
Device Downloading, Connectivity, and Interoperability
Manual recording of glucose data is fraught with inaccuracies (115). Most meters can be downloaded, usually via a tethering cable or wireless connection, either by the patient or healthcare provider. Each glucose monitoring device generally works with its own proprietary management software. However, several programs (Tidepool, Glooko/Diasend, Carelink by Medtronic, Accu-chek) are capable of downloading and organizing data for multiple different devices (116). Reports are standardized across all device downloads, facilitating efficient and actionable patient and healthcare provider review. These programs also facilitate population health and telehealth strategies (discussed below). The Nightscout Project is a crowd sourced application provides a free mobile technology platform for patients who want to access their devices in real time on any mobile device (117). In actuality, a minority of patients with type 1 diabetes download and review their own glucose monitoring data, and few download routinely (118). In the Type 1 Diabetes Exchange Registry, 65% never download their meter, and only 12% download at least monthly (119). There are few data among patients with type 2 diabetes, but there is no reason to believe that the frequency is any better.
Direct connectivity of blood glucose or CGM levels to cell phones or other devices not only improves data integrity but may also simplify the assimilation of glucose levels with other data such as insulin use, carbohydrate intake, and activity levels for the purpose of facilitating insulin dose adjustments in real time or retrospectively. Cell phone connectivity may also improve communication with providers. A few meters with direct cellular capability are now available. Miniaturized glucometers that directly plug into a smart phone also allow users to consolidate devices. Devices with direct cellular or Bluetooth connections may be paired with apps that facilitate collection, communication, and analysis of a variety of data and provide tools for education (such as nutrition information) at the point of care.
Currently, no devices yet allow control of insulin delivery from personal mobile devices, in part due to regulatory and security concerns. However, there are several efforts under way, including a recent collaboration between Insulet's Omnipod DASH Insulin Management System and Samsung (120). Until recently, insulin pumps have been cleared by the FDA as stand-alone devices or as a complete system. Recently, a new regulatory pathway was developed for alternate controller enabled (ACE) infusion pumps, which means that it can be operated in conjunction with interchangeable components, particularly CGMs (121). In 2019, the FDA approved the first such devices (Tandem t:Slim X2 and Omnipod DASH system).
Diabetes Apps
A variety of stand-alone smart phone applications that support SMBG are also available. Most provide information, track SMBG data (usually manually entered), some allow insulin or carbohydrate documentation, facilitate carbohydrate or calorie counting, promote weight loss, track or promote physical activity, enhance medication adherence, and use motivational or self- efficacy approaches, and a few provide an insulin dosing calculator (111). Simple apps provide information or tracking functions while more sophisticated approaches incorporate gamin theory and machine learning approaches that learn from the user's previous experiences to optimize interactions. Apps have shown limited magnitude and sustainability of effect due to a variety of factors, including user fatigue, require continuous data entry (eg. most apps do not connect directly with a glucose meter), and lack of integration with the health care team. Moreover, most apps have not been evaluated by the FDA or other regulatory agencies. Data privacy is also a concern, as no federal regulations currently prevent app developers from disclosing data to third parties. Most apps (81% in one survey of Android apps) do not have privacy policies, and of those that do, 49% share user data with third parties (122). Expert groups have recently developed policy or guidance statements that may lead to standardization and increased functionality (123,124). Most recently, the International Diabetes Federation-Europe published a position statement which provided recommendations for individuals with diabetes, health care providers, policy makers, and app developers. Of note, the statement recommends that app developers should consult with patients and patient groups, evaluate effectiveness before launching, ensure appropriately tailored content for persons with diabetes as well as for healthcare professionals, consider interoperability with mobile devices, healthcare devices, and electronic medical records systems, build upon existing scientific research, and utilize scientific committees to support development (125).
Efficacy
While the data are still evolving with respect to mobile diabetes applications, several systematic reviews and meta-analyses are available. In 2016, Hou and colleagues conducted a meta-analysis of 14 randomized controlled trials among patients with type 2 diabetes (126). There were insufficient data to analyze type 1 diabetes studies. The reported mean reduction in A1C was 0.49% (95% CI 0.30-0.68), with moderate grade evidence. Effect size was larger in younger patients and those with healthcare provider feedback. A similar, modest reduction in A1C was also reported in other meta-analyses (127,128).
The Agency for Healthcare Research and Quality published a systematic review of comparative effectiveness studies assessing apps or programs available through a mobile device for the purpose of diabetes self-management (129). For type 1 diabetes, 6 apps were identified, 3 of which were associated with improvement in A1c, 2 of which were associated with improvement in hypoglycemia. Five apps for patients with type 2 diabetes were identified, 3 of which were associated with improvement in A1c. Efficacy is variable, in part because app features vary but also because apps are often studied as part of a multi-component intervention, making it difficult to assess individual elements, particularly the effect of additional health care provider support.
In another meta-analysis, investigators developed a taxonomy system to classify apps in order to facilitate understanding of the contribution of individual functions to outcomes (130).
Functions were classified according to functional (technical) characteristics (log, structured display, education, personalized feedback, and communication) and clinical features (glucose monitoring, medication management, lifestyle modification, complication prevention, and psychosocial care). A separate risk axis was specified based upon whether the app provided communication or administrative functions (low risk), health management (decision support and medication management—potential risk), or medical device interface (high risk). All 12 apps included a glucose monitoring function. Three apps were classified as high risk. The mean A1C reduction was 0.48% (95% CI 0.19-0.77) across studies, but was limited to patients with type 2 diabetes and the overall quality of evidence was low. A1C reduction was greater when the intervention included a complication prevention module, a structured display, no clinical decision-making function, and wireless (vs manual) entry. The quality of evidence for severe hypoglycemia was low.
Other researchers have focused on identifying standard evidence-based features that should be included in diabetes apps. Adu and colleagues performed a systematic review that identified 11 studies, all of which incorporated glucose monitoring functions but only 7 included data analytic functions, 6 incorporated education, and 6 provided reminders (131). A1c reduction was associated with education functions, but none of the apps reported all of the developmental steps assessed. Martinex-Millana and colleagues assessed whether commercially available apps display evidence based features known to positively affect type 1 diabetes management using an adapted taxonomy that addressed 10 basic themes including personalization, family support, agenda (appointments), data recording, insulin bolus calculation, data management, interaction with others, tips/support, reminders, and rewards. None of the 80 apps met all of the criteria for an "ideal" app, but the most common features were personalization and data recording and the least common were agenda and rewards (132).
Usability
In a systematic review of 20 studies, only one third of the 20 apps met the authors' health literacy standards (133). Usability was measured in 7 studies through satisfaction surveys from patients and experts, and ranged from 38-80%. The most common usability problems were multi-step tasks, limited functionality, and poor system navigation.
Recently, a Mobile App Rating Scale was utilized to assess the top free (without subscription), patient directed, English language apps in iTunes and Google Play for "diabetes" and "diabetes management" (134). Most apps were rated by 3 reviewers to be high quality for a single task but only 4/89 apps integrated all diabetes management tasks (physical activity, nutrition, blood glucose monitoring, medication usage, health feedback, and education). The top scoring apps were Tactio Health: My Connected Health Logbook, and ACCU-CHEK 360 Diabetes Mgmt).
In a similar approach, Brzan and colleagues evaluated 65 freely available apps assessed by 3 expert reviewers for published criteria promoting diabetes self-management (SMBG, medication, nutrition, exercise, weight). Most apps (56/65) did not meet minimal requirements or did not function properly. Only 9 apps were determined to be versatile and useful for diabetes self-management (135).
In the AHRQ review referenced above, only 1 out of 6 apps for type 1 diabetes and 1 out of 5 apps for type 2 diabetes demonstrated acceptable usability (129).
Decision Support
The use of pattern management software improves health care provider efficiency and accuracy in identifying needed therapeutic adjustments (136,137). Software programs provide graphs or charts and may in some cases provide dosing advice, either for the healthcare provider or directly to the patient.
Insulin Dosing Calculators
Insulin dosing calculators have been used for years as a means of incorporating glucose measures into routine practice, largely in concert with continuous insulin infusion pumps. While numerous apps have become available for bolus insulin calculation and basal insulin titration, it is important to note that only a few have been formally evaluated and approved by regulatory agencies. In addition, many still require manual data entry, few integrate within existing electronic medical records, and published evidence for efficacy is limited (138). As with automated insulin delivery, cybersecurity is critical in order to avoid the potential for unauthorized adjustments to insulin dosing. Therefore, only evidence-based applications with regulatory approval should be used. All approved insulin calculators or dose titration apps require a prescription or need to be set up by a healthcare provider.
BOLUS CALCULATORS
Bolus calculators are known to substantially improve dosing accuracy and glycemic control in outpatients with type 1 diabetes (139,140,141). Subjects with diabetes feel more confident using the calculator and prefer it to manual calculations of insulin doses. Bolus calculators might be particularly helpful for patients with poor numeracy. A number of stand-alone smart-phone apps for bolus insulin calculation have been developed but as noted above, their safety and efficacy remains a concern (142,143). Though algorithms typically incorporate the current glucose level, active insulin time, and carbohydrate intake, some do not account for activity or illness, and none account for protein or fat content, which may also impact insulin dose responses. Moreover, applications that improve the accuracy of carbohydrate counting, which is a major source of error (regardless of educational level), are desirable (144). A review of several approved systems is presented here.
- Accuchek Aviva Expert
: This system (Roche) was a stand-alone glucose meter with a built- in bolus calculator that was replaced by an Accu-Chek Connect meter that wirelessly transmits data to a smartphone app or web portal with a built-in FDA and CE approved bolus calculator. Bolus advice includes adjustments for significant health events such as exercise or illness. The Bolus Insulin Advisor needs to be set up by the health care provider. The app also provides the ability to share data with others via automatic SMS messaging as well as with healthcare professionals via an online portal (145). The Accu-Chek 360 View software can be used to view and process glucose, carbohydrate, and insulin doses to inform management decisions. The 26-week Accu-Chek Bolus Advisor Control and Usability Study (ABACUS) randomly assigned over 200 patients with diabetes to use of the bolus calculator or usual care (146). Patients using the bolus advisor were more likely than controls to achieve >0.5% A1c reduction (56 vs. 34%, p<0.01).
- Freestyle InsuLinx
: This system (Abbott) has a touchscreen interface and contains a bolus calculator that is available in fixed meal or flexible meal dosing modes (147). The bolus calculator incorporates correction dosing as well as an active insulin time. The bolus calculator is only available outside of the U.S., but a simplified version without the bolus advisor, which allows the patient to record insulin and glucose data is FDA approved. There is an online portal FreeStyle Auto-Assist DM, for health care professionals to access and process data.
- Dario
: The Dario (LabStyle Innovations) meter is a small glucose monitoring device that directly plugs into a smart phone, where glucose data is stored in the cloud via an app (148). The app provides access to an insulin bolus calculator (available in Europe) and carbohydrate counting tool and facilitates real-time data sharing with family and caregivers and activity trackers. The system will also send a text message plus GPS coordinates to designated individuals in the event of hypoglycemia. There are no published efficacy studies but the device has been FDA and CE approved.
- Diabeo
is an app that consists of an e-diary, bolus calculator (incorporating the current glucose, carbohydrate intake, and activity) and basal insulin calculator. Diabeo is approved in France and provides an automated algorithm for dose titration and results in a significant A1c reduction (149).
- Diabetes Interactive Diary
: This is a CE approved app with a built-in bolus calculator that communicates with the health care provider and contains a photographic food atlas to assess the nutritional content of a meal and has been found to reduce the time required for education (150), reduce hypoglycemia (151), and improve quality of life (150).
INSULIN DOSING CALCULATORS
More recently insulin dosing calculators have been approved to recommend ongoing adjustments in therapy, either for titration or for mealtime insulin calculations. Unfortunately, efficacy and safety studies are not currently available for most apps. Most basal insulin titration apps account only for fasting glucose measures and not overnight trends. However, the following are approved by the FDA or in Europe.
- The Diabetes Insulin Guidance System
(Hygieia) uses a d-Nav glucose monitor with built-in insulin dosing calculator that is set up by the healthcare provider to titrate the insulin doses based upon historical glucose values and insulin doses. The system is approved in Europe but not the US. Efficacy has only been demonstrated with a single observational study, in which users demonstrated a reduction in A1C and hypoglycemia compared to baseline (152).
- My Dose Coach
(Sanofi Aventis) is an app that was FDA cleared in 2017 to optimize basal insulin dosing (153). The healthcare provider sets up the individualized dose plan on a separate provider portal and the user activates the app with a verification code, received via text message.
- Insulia
(Voluntis, in partnership with Sanofi and Livongo) is a software management system with accompanying app available on a prescription basis. It is FDA approved for detemir, glargine U100 and Glargine U300 dosing and is also CE approved (154).
- Go Dose
(Eli Lilly) is an app (patient version and Go Dose Pro for healthcare providers) for adjusting prandial lispro one meal at a time and was FDA approved in 2017. The app is only available by prescription (155).
- iSage-Rx
is an FDA approved app for basal insulin titration in type 2 diabetes and provides customizable algorithms (156). There are no published studies in the literature.
- InPen
is a smartpen that is compatible with rapid-acting insulins via refillable cartridges (157). The pen links to a diabetes management app as well as the Accu-Chek Connect glucometer via Apple Health. The app provides prandial and correction insulin dose recommendations for fixed meal or flexible meal dosing strategies and also provides advice for treatment of hypoglycemia including reverse insulin calculations. The app allows customizable targets, insulin:carbohydrate ratios, and correction factor/sensitivity by time of day. All actual insulin doses are communicated to the app via Bluetooth connection so that actual doses can be reviewed and compared with bolus advice. The device allows for half- unit dosing.
Integrated Digital Approaches
Integrated telemedicine approaches have received increasing attention as a means of providing cost-effective care (158,159,160). Some results of telemonitoring studies have been somewhat disappointing, perhaps due to delayed or infrequent feedback to patients (161,162). Approaches in which real-time feedback is provided have shown more promising results. In fact, the benefit from these approaches may not necessarily derive from adjustment of medications such as insulin, but from a combination of increased self-efficacy and motivation as a result of better patient-provider communication and support (115).
- WellDoc
: The WellDoc system consists of patient coaching as well as provider clinical decision support. Patients view data via their mobile device through a web portal and receive automated text messaging responses that are tailored to the data. Diabetes educators or other providers may view the data and send supplemental feedback as well. In a 12 month cluster randomized trial of patients with type 2 diabetes, the system was shown to reduce A1c by 1.2% vs. usual care (163).
- Diabeo:
As noted above, the Diabeo system resulted in slightly better A1c reduction of 0.91% when used with biweekly teleconsultation vs. 0.67% without teleconsultation when compared to placebo (149).
- Livongo In Touch
is a cellular enabled color touchscreen platform with a built-in glucose meter that directly uploads data to the cloud, without a need for Wi-Fi, Blue-tooth, or tethering cables (164). It provides immediate personalized feedback to the patient based upon glucose levels, tailored text messages, and access to certified diabetes educators in real time. A patient portal also allows for integration of data from multiple sources including third party nutrition and exercise apps. Providers can access dashboard reports for population and telehealth programs. Test strips are unlimited with a monthly service fee. The system is accredited by the American Association of Diabetes Educators and FDA approved. In a retrospective study of 4,544 patients with diabetes, users were 18% less likely to experience hypoglycemia and experienced a 16% reduction in hyperglycemia (165). In a randomized study of 330 adults with type 2 diabetes, Livongo participation alone (connected meter with two-way messaging, unlimited test strips and access to certified diabetes educators [CDEs]) was associated with a reduction in A1c from 8.5 to 7.5% (p=0.01) (166). Additional intensive coaching was associated with greater mean weight loss and additional glucose reductions at the expense of greater costs.
- Telcare
is another cellular enabled glucose meter that automatically uploads glucose data to a cloud and can be shared with family and health care providers. Users receive automated personalized messages in response to specific glucose values and providers can also send custom messages in real-time. Data can be accessed with the Diabetes Pal app or through an online portal. In a pragmatic clinical trial of 450 non-insulin requiring patients with type 2 diabetes randomized to no SMBG, once daily SMBG, or Telcare system, there was no difference in A1C or quality of life among groups (167). However, when utilized within other programs that provide robust patient feedback, results may differ.
- Glucommander Outpatient
(Glytec) is an FDA approved glucose management and titration platform that analyzes glucose patterns and provides insulin titration advice to healthcare providers. In a retrospective study with historical control of 46 patients, the A1c was reduced from 10.2 to 7.8% at 6 months, and 7.2% at 12 months (p<0.00001) (168).
- Onduo
, a joint venture between Sanofi Aventis and Verily, is a virtual diabetes clinic that utilizes the Telcare cellular glucometer with unlimited testing supplies, A1c kits, Dexcom CGM (in select individuals) and access to health coaches, CDEs, and physicians on staff via a dedicated app to provide a tailored program for diabetes self-management (169). The program has partnered with the American Association of Diabetes Educators but there are currently no published studies.
- MySugr:
MySugr is an app that utilizes data imported from linked meters or manual entry and supports patients in American Association of Diabetes Educators designated 7 health behaviors. The app is approved by the FDA and contains a bolus calculator that is approved only by the CE. It provides messages based upon behavioral psychology and game theory. The associated mySugr Bundle also provides unlimited test strips and immediate access to CDEs, who utilize population management resources and pattern recognition software to identify and monitor patients at high risk. Favorable glucose control and patient satisfaction outcomes were reported in retrospective and observational studies (170).
- One Drop
is a diabetes platform that integrates a Bluetooth enabled glucose meter with unlimited testing supplies, coaching programs, and mobile app. In a retrospective study of 367 patients with type 1 diabetes and 921 patients with type 2 diabetes, it was associated with a 1.1 to 1.3% reduction in A1c, with better A1c reductions in type 2 diabetes and among users who recorded food intake within the app (171). In a prospective nonrandomized study (N=146) use of the coaching function was associated with incrementally greater A1c reductions (172).
- DreaMed Advisor Pro
: This system analyzes insulin pump and CGM download information and provides dose adjustment advice based upon an algorithm that was developed by specialists. Healthcare providers can access the algorithm via an interface with Glooko or Tidepool. In a recent study, agreement between 26 physicians on insulin adjustments recommended for 15 patient downloads was less than 50% but was not significantly different from the automated algorithm, which was less than or equal to that recommended by the experts (173). A randomized controlled trial is ongoing.
Although there are a plethora of apps available, the ultimate choice should be individualized to the needs of the patient. Those patients only needing a resource that assists with carbohydrate counting can be referred to common apps like MyFitnessPal or Calorie King. For glucose monitoring, apps that require manual entry of data should be minimized as they are not likely to be utilized long-term. Universal platforms (such as Glooko, Tidepool, Carelink, Diasend) that can download multiple devices can increase clinic efficiency. Where possible, patients should be invited to directly link with their clinic (most devices have to be manually downloaded, but a few that communicate with mobile apps (such as Dexcom, Freestyle Libre, and T-slim) can automatically and continually link to the clinic account, removing the need for a computer. This is particularly useful for telehealth visits. Smart insulin pens provide assistance with insulin dosing and can also be downloaded using some universal platforms. The major limitation of these applications however, is that patient generated data generally does not integrate within the EMR in a meaningful way. Some opportunities exist with the integration of Apple Health Kit and Samsung S-Health which can transmit data from a variety of apps but this process requires multiple steps and can be cumbersome (174,175). Importantly, none of these mobile health tools replace frequent patient contact and feedback.
BIOMARKERS OF GLYCEMIC CONTROL
Hemoglobin A1c (A1C)
A1C is the best biomarker indicator of glycemic control over the past 2-3 months due to strong data predicting complications (1,2). In addition, the American Diabetes Association has recommended its use for the diagnosis of diabetes (1).
Hemoglobin A1c refers to the nonenzymatic addition of glucose to the N-terminal valine of the hemoglobin beta chain. Assays are based upon charge and structural differences between hemoglobin molecules (176,177). Therefore, variants in hemoglobin molecules may lead to analytic interferences. It should be noted that some homozygous hemoglobin variants (HbC or HbD, or sickle cell disease) also alter erythrocyte life span and therefore, even if the assay does not show analytic interference, other methods of monitoring glycemia should be utilized, as HbA1c will be falsely low. Individual assay interferences are available at the National Glycohemohemoglobin Standardization Program website: www.ngsp.org/interf.asp (178).
Several commercial home monitoring kits are also available (179). The two reference methods used to standardize A1c levels are 1) HPLC and electrospray ionization mass spectrometry or 2) a two-dimensional approach using HPLC and capillary electrophoresis with UV-detection (180). A brief summary of assay methods is described below.
-
HPLC methods utilize the fact that glycated hemoglobin has a lower isoelectric point and migrates faster than other hemoglobin components. As such it has variable interference with hemoglobinopathies that alter the charge of the molecule (such as HbF and carbamylated Hb), but these may be revealed through individual inspection of the chromatograms.
-
Boronate affinity methods are based upon glucose binding to m-aminophenylboronic acid and measures glycation on the N-terminal valine on the beta chain but also glycation at other sites. There is minimal interference from hemoglobinopathies but this assay is not widely available.
-
Immunoassays make use of antibody binding to glucose and N-terminal amino acids on the beta chain and therefore may be affected by hemoglobinopathies with structural changes at these sites, including HbF but not HbE, HbD, or carbamylated Hb. Some newer assays have attempted to correct for these interferences.
-
Enzymatic methods lyse whole blood, releasing glycated N-terminal valines which are detected using a chromoagenic reaction and are not affected by hemoglobin variants.
An Organization with links to governmental regulatory agencies, the National Glycohemoglobin Standardization Program (NGSP) (<http://www.ngsp.org/news.asp >), evaluates every laboratory and home test for A1C, sets accuracy standards, and certifies which methods meet their standards (181). The trend in industry is for monitors to become increasingly more accurate and the trend in regulatory organizations is to require increasing accuracy for ongoing certification.
A1C is an analyte found within red blood cells, comprised of glycated Hemoglobin. The glycation gap (formerly known as the glycosylation gap) (GG), based on fructosamine measurement, and the Hemoglobin Glycation Index (HGI), based on mean blood glucose, are two indices of between-individual differences in glycated hemoglobin adjusted for glycemia. GG is the difference between the measured A1C test and the A1C test result predicted from serum fructosamine testing based on a population regression equation of A1C on fructosamine (182). and HGI is the difference between the measured A1C test and A1C results predicted from the mean blood glucose level (calculated from self-monitored blood glucose tests) based on a population regression equation of A1C tests on mean blood glucose levels (183). These two indices are consistent within an individual over time and reflect an inherent tendency for an individual's proteins to glycate (184,185). Patients with high GG and HGI indices might have falsely high A1C test results and might also be at increased risk of basement membrane glycosylation and development of microvascular complications. Whether between-individual biological variation in Hemoglobin A1c is an independent risk factor, distinct from that attributable to mean blood glucose or fructosamine levels, for diabetic microvascular complications is controversial (186).
Because the A1C test is supposed to reflect the mean level of glycemia, attempts have been made to correlate this widely-accepted measure with empirically measured mean blood glucose levels. In 2008, the A1c-Derived Average Glucose (ADAG) study compared HbA1c and continuous glucose monitoring derived mean glucose and 7-point glucose profiles among 507 patients with type 1 and type 2 diabetes and without diabetes from 10 international centers to derive an estimated average glucose (eAG) from A1C levels using the following equation: eAG(mg/dl)=(28.7*HbA1c)-46.7 (Table 6).
Table 6.
A1C and Estimated Average Glucose
View in own window
A1C (%) | eAG (mg/dl) | eAG (mmol/l) |
---|---|---|
5 | 97 | 5.4 |
6 | 126 | 7.0 |
7 | 154 | 8.6 |
8 | 183 | 10.2 |
9 | 212 | 11.8 |
10 | 240 | 13.4 |
11 | 269 | 14.9 |
12 | 298 | 16.5 |
Several lines of evidence support this disconnect from a tight correlation between mean glycemia and A1C levels. First, improvements in mean glycemia may not necessarily be reflected by improvements in A1C in intensively treated patients (187). A1C does not reflect short-term changes in glucose control, and therefore can be misleading where there have been recent changes in the clinical condition. In addition, glucose fluctuations, compared to chronic sustained hyperglycemia, have been shown to exhibit a more specific triggering effect on oxidative stress and endothelial function (188,189). Glycemic variability cannot be assessed by a global measure of mean glycemia, such as A1C, but requires multiple individual glucose values, such as what can be obtained from continuous glucose monitoring or from seven-point- per-day (or greater) self-glucose testing. Third, A1C does not permit specific adjustments in therapy, particularly among patients requiring insulin titration. Finally, A1C reliability may be affected several conditions that alter red blood cell lifespan and its use in these circumstances can be misleading. A comparison of the features and limitations in glucose markers is presented in Table 7 (190,191,192).
Table 7.
Comparison of Markers of Glycemic Control
View in own window
Biomarker mechanism | Interval of time reflecting glucose control | Cautions/Interferences | |
---|---|---|---|
A1C | Hemoglobin glycation | 3 months | Hemoglobinopathy (↑/↓*) Decrease in RBC survival (hemolysis, splenomegaly, pregnancy, drugs) (↓) Increase in RBC survival (Erthyropoietin, iron replacement) (↑) Transfusion (↓) |
Fructosamine | Protein glycation | 2 weeks | Conditions resulting in hypoproteinemia (severe cirrhosis, nephrotic syndrome, enteropathy) (↓) High dose Vitamin C, severe hyperbilirubinemia/uremia/ hypertriglyceridemia (↑) |
1,5-AG | Renal clearance | 1 week | Chronic kidney disease (stage 4, 5) (↓) Glucosuria (pregnancy, renal tubular disorders SGLT2 inhibitors) (↓) Advanced cirrhosis (↓) High soy diet (↑) |
Ethnic differences in A1C have also been reported (193). For example, recent data from the Type 1 Diabetes Exchange demonstrates a 0.4% higher A1C at a given mean glucose among black patients compared to white patients with type 1 diabetes, but no effect of race on glycated albumin or fructosamine (194). However, NHANES data do not demonstrate an effect of ethnicity on the association between A1C and retinopathy (195). Data from the ARIC study demonstrated that A1C, fructosamine, glycated albumin, and 1,5-AG were consistent with residual hyperglycemia among blacks compared to whites, and the prognostic value for incident cardiovascular disease, end stage renal disease and retinopathy were similar by race (196). It should be noted that the range of available A1C was relatively narrow in NHANES and ARIC, and further data across an expansive range is needed. In relation to CGMs, utility of A1C is further enhanced when used as a complement to glycemic data measured by CGM (13). Other biomarkers are becoming more widely used, however, A1C remains the most common biomarker. Other measures of average glycemia such as fructosamine and 1,5-anhydroglucitol are available, but their translation into average glucose levels and prognostic significance are not as clear as for A1C (1).
Fructosamine
A short to medium-term marker (reflecting the average glucose control over the past few weeks) may be useful for determining control over a period of days to weeks since A1C does not reflect recent changes in glucose control. Alternate markers may also be useful in patients with discrepant A1C and self-monitored blood glucose readings as well as patients with other hematologic conditions known to affect A1C. Fructosamine is a term that refers to a family of glycated serum proteins and this family is comprised primarily of albumin and to a lesser extent, globulins, and to an even lesser extent, other circulating serum proteins. No product exists for home use that measures serum fructosamine. A home blood fructosamine monitor, Duet Glucose Control System, was marketed in the early 2000′s and then withdrawn from the market. No subsequent home fructosamine test has been available since then. Randomized controlled trials have reported inconsistent effects of frequent monitoring on A1C lowering, possibly due to differences in execution of therapeutic interventions (197,198). Serial monitoring of short-term markers may also facilitate timely elective surgery in patients whose procedure is delayed due to an elevated A1C. In a recent study, fructosamine was a better predictor of post-operative complications in patients undergoing primary total joint arthroplasty (199).
GLYCATED ALBUMIN
The largest constituent of fructosamine is glycated albumin. Several investigators and companies are developing portable assays for glycated albumin to assess overall control during periods of rapidly changing glucose levels. In these situations, an A1C test may change too slowly to capture a sudden increase or decrease in mean glycemia. The components of the necessary technology appear to be in place to build a commercial instrument for home testing of glycated albumin. However, there is no randomized controlled trial showing that the measurement of glycated albumin improves outcomes. In the ARIC study, fructosamine, glycated albumin, and 1,5-AG were associated with incident diabetes, even after adjustment for baseline A1C and fasting glucose. In the ARIC study, both fructosamine and glycated albumin predicted incident retinopathy and nephropathy, even after adjusting for A1C (200). However, in adults with severe chronic kidney disease, none of the markers, including A1C, fructosamine, or glycated albumin were very highly correlated with fasting glucose, and there did not appear to be an advantage of one marker over another (201). In addition, baseline glycated albumin and fructosamine were associated with cardiovascular outcomes over a 20 year follow-up period after adjusting for other risk factors, but the overall magnitude of associations was similar to A1C (202). In the Diabetes Control and Complications Trial (DCCT), glycated albumin had a similar association with retinopathy and nephropathy as A1C, but the combination of both markers provided even better prediction (203).
Short-term markers are also of interest for use in pregnancy, where glucose levels are changing more quickly than can be reflected by A1C. Unfortunately, glycated albumin does not predict gestational diabetes more effectively than A1C or fasting glucose (204). However, other preliminary data suggests that glycated albumin may be a better predictor of pregnancy complications than A1C (205).
1,5-Anhyroglucitol
The aforementioned biomarkers for measuring glycemic control, (A1C, fructosamine, and glycated albumin) only reflect mean levels of glycemia. These measures can fail to portray hyperglycemic excursions if they are balanced by hypoglycemic excursions. Plasma 1,5- anhydroglucitol (1,5-AG) is a naturally occurring dietary monosaccharide, with a structure similar to that of glucose (Figure 12). This analyte has been proposed as a marker for postprandial hyperglycemia (206). An automated laboratory grade assay named Glycomark is approved in the U.S. for measuring 1,5-AG as a short-term marker for glycemic control. A similar laboratory assay has been used in Japan. During normoglycemia, 1,5-AG is maintained at constant steady-state levels because of a large body pool compared with the amount of intake and because this substance is metabolically inert. Normally, 1,5-AG is filtered and completely reabsorbed by the renal tubules. During acute hyperglycemia when the blood glucose levels exceed 180 mg/dl, which is the renal threshold for spilling glucose into the urine, serum 1,5-AG falls. This fall occurs due to competitive inhibition of renal tubular reabsorption by filtered glucose. The greater the amount of glucose in renal filtrate (due to hyperglycemia), the less 1,5-AG is reabsorbed by the kidneys. The 1,5-AG levels respond sensitively and rapidly to rises in serum glucose and a fall in the serum level of this analyte can indicate transient elevations of serum glucose occurring over as short a period as a few days. Measurement of 1,5-AG can be useful in assessing the prior 1-2 weeks for: 1) the degree of postprandial hyperglycemia; and 2) the mean short-term level of glycemia. This assay might prove useful in assessing the extent of glycemic variability that is present in an individual with a close-to-normal A1C level, but who is suspected to be alternating between frequent periods of hyperglycemia and hypoglycemia. In such a patient, the 1,5-AG level would be low, which would indicate frequent periods of hyperglycemia, whereas in a patient with little glycemic variability, the 1,5-AG levels would not be particularly depressed because of a lack of frequent hyperglycemic periods. In the ARIC study, 1,5-AG was associated with severe hypoglycemia after adjustment for other variables, an observation which is consistent with the role of 1,5-AG in reflecting glycemic variability, a known risk factor for hypoglycemia (207).
Longitudinal data from the ARIC study showed that 1,5-AG was associated with ESRD over a 19-year follow-up period, but the relationship was no longer significant after adjusting for glucose control with other markers (208). Among participants with diabetes and A1C <7%, each 5 mcg/mL decrease in 1,5-AG was associated with an increase in dementia risk by 16%, and at A1C >7%, there was also a significant association over a median 21-year follow-up period (209). There was also an association of 1,5-AG and cardiovascular outcomes in ARIC, which persisted, though were attenuated after adjusting for A1C (210). Therefore, it is not yet clear whether 1,5-AG, as a measure of glucose excursions, provides incremental value beyond A1C for predicting long-term complications.
Figure 12.
Structure of glucose (left) and 1,5 anhydroglucitol (right)
CONCLUSIONS
Many new types of technology are increasingly being developed and applied to fight diabetes and its complications. New technologies will improve the lives of people with diabetes by measuring glucose and other biomarkers of glycemic control and linking glucose levels with insulin delivery to improve the lives of people with diabetes.
REFERENCES
- 1.
-
American Diabetes Association Position Statement: Standards of Medical Care in Diabetes Care 2019 42:(Suppl 1) S1-S80.
- 2.
-
Bailey TS, Grunberger G, Bode BW, Handelsman Y, Hirsch IB, Jovanovič L, Roberts VL, Rodbard D, Tamborlane WV, Walsh J., American Association of Clinical Endocrinologists (AACE). American College of Endocrinology (ACE). American Association of Clinical Endocrinologists and American College of Endocrinology 2016 Outpatient Glucose Monitoring Consensus Statement. Endocr Pract. 2016;22(2):231–61. [PubMed: 26848630]
- 3.
- 4.
-
Peters AL, Ahmann AJ, Battelino T, Evert A, Hirsch IB, Murad MH, Winter WE, Wolpert H. Diabetes Technology-Continuous Subcutaneous Insulin Infusion Therapy and Continuous Glucose Monitoring in Adults: An Endocrine Society Clinical Practice Guideline. J Clin Endocrinol Metab. 2016;101(11):3922–3937. [PubMed: 27588440]
- 5.
-
Gross TM, Bode BW, Einhorn D, Kayne DM, Reed JH, White NH, Mastrototaro JJ. Performance evaluation of the MiniMed continuous glucose monitoring system during patient home use. Diabetes Technol Ther. 2000;2:49–56. [PubMed: 11467320]
- 6.
-
Welsh J, Kaufman FR, Lee SW. Accuracy of the Sof-Sensor Glucose Sensor with the iPro Calibration Algorithm. J Diabetes Sci Technol. 2012;6(2):475–476. [PMC free article: PMC3380794] [PubMed: 22538161]
- 7.
- 8.
-
Bailey T, Bode BW, Christiansen MP, Klaff LJ, Alva S. The Performance and Usability of a Factory-Calibrated Flash Glucose Monitoring System. Diabetes Technol Ther. 2015;17(11):787–94. [PMC free article: PMC4649725] [PubMed: 26171659]
- 9.
- 10.
-
Ólafsdóttir AF, Attvall S, Sandgren U, Dahlqvist S, Pivodic A, Skrtic S, Theodorsson E, Lind M. A Clinical Trial of the Accuracy and Treatment Experience of the Flash Glucose Monitor FreeStyle Libre in Adults with Type 1 Diabetes. Diabetes Technol Ther. 2017;19(3):164–172. [PMC free article: PMC5359691] [PubMed: 28263665]
- 11.
-
Miele A, Weiland K, Dungan KM. Clinical outcomes associated with referral-based continuous glucose monitoring using a central standardized interpretation strategy. Diabetes Technol Ther. 2012;14(9):765–71. [PubMed: 22928614]
- 12.
-
Bergenstal RM, Ahmann AJ, Bailey T, Beck RW, Bissen J, Buckingham B, Deeb L, Dolin RH, Garg SK, Goland R, Hirsch IB, Klonoff DC, Kruger DF, Matfin G, Mazze RS, Olson BA, Parkin C, Peters A, Powers MA, Rodriguez H, Southerland P, Strock ES, Tamborlane W, Wesley DM. Recommendations for standardizing glucose reporting and analysis to optimize clinical decision making in diabetes: the Ambulatory Glucose Profile (AGP). Diabetes Technol Ther. 2013;15(3):198–211. [PubMed: 23448694]
- 13.
-
Battelino T, Danne T, Bergenstal RM, et al. Clinical Targets for Continuous Glucose Monitoring Data Interpretation: Recommendations From the International Consensus on Time in Range. Diabetes Care. 2019;42(8):1593–1603. [PMC free article: PMC6973648] [PubMed: 31177185]
- 14.
-
Keenan DB, Mastrototaro JJ, Zisser H, Cooper KA, Raghavendhar G, Lee SW, Yusi J, Bailey TS, Brazg RL, Shah RV. Accuracy of the Enlite 6-day glucose sensor with Guardian and Veo calibration algorithms. Diabetes Technol Ther. 2012;(14):225–231. [PubMed: 22145851]
- 15.
-
Christiansen M, Bailey T, Watkins E, Liljenquist D, Price D, Nakamura K, Boock R, Peyser T. A new-generation continuous glucose monitoring system: improved accuracy and reliability compared with a previous-generation system. Diabetes Technol Ther. 2013;15(10):881–8. [PMC free article: PMC3781114] [PubMed: 23777402]
- 16.
-
Luijf YM, Mader JK, Doll W, Pieber T, Farret A, Place J, Renard E, Bruttomesso D, Filippi A, Avogaro A, Arnolds S, Benesch C, Heinemann L, DeVries JH. AP. @home consortium. Accuracy and reliability of continuous glucose monitoring systems: a head-to-head comparison. Diabetes Technol Ther. 2013;15(8):722–7. [PMC free article: PMC3746288] [PubMed: 23650900]
- 17.
-
Deiss D, Bolinder J, Riveline JP, Battelino T, Bosi E, Tubiana-Rufi N, Kerr D, Phillip M. Improved glycemic control in poorly controlled patients with type 1 diabetes using real- time continuous glucose monitoring. Diabetes Care. 2006;29:2730–2732. [PubMed: 17130215]
- 18.
-
Juvenile Diabetes Research Foundation Continuous Glucose Monitoring Study Group. Tamborlane WV, Beck RW, Bode BW, Buckingham B, Chase HP, Clemons R, Fiallo- Scharer R, Fox LA, Gilliam LK, Hirsch IB, Huang ES, Kollman C, Kowalski AJ, Laffel L, Lawrence JM, Lee J, Mauras N, O'Grady M, Ruedy KJ, Tansey M, Tsalikian E, Weinzimer S, Wilson DM, Wolpert H, Wysocki T, Xing D. Continuous glucose monitoring and intensive treatment of type 1 diabetes. N Engl J Med. 2008;359(14):1464–76. [PubMed: 18779236]
- 19.
-
Battelino T, Phillip M, Bratina N, Nimri R, Oskarsson P, Bolinder J. Effect of continuous glucose monitoring on hypoglycemia in type 1 diabetes. Diabetes Care. 2011;34(4):795–800. [PMC free article: PMC3064030] [PubMed: 21335621]
- 20.
-
Bolinder J, Antuna R, Geelhoed-Duijvestijn P, Kröger J, Weitgasser R. Novel glucose- sensing technology and hypoglycaemia in type 1 diabetes: a multicentre, non-masked, randomised controlled trial. Lancet. 2016;388(10057):2254–2263. [PubMed: 27634581]
- 21.
-
Reddy M, Jugnee N, El Laboudi A, Spanudakis E, Anantharaja S, Oliver N. A randomized controlled pilot study of continuous glucose monitoring and flash glucose monitoring in people with Type 1 diabetes and impaired awareness of hypoglycaemia. Diabet Med. 2017;35(4):483–90. [PMC free article: PMC5888121] [PubMed: 29230878]
- 22.
-
Bergenstal RM, Tamborlane WV, Ahmann A, Buse JB, Dailey G, Davis SN, Joyce C, Peoples T, Perkins BA, Welsh JB, Willi SM, Wood MA. STAR 3 Study Group. Effectiveness of sensor-augmented insulin-pump therapy in type 1 diabetes. N Engl J Med. 2010;363(4):311–20. [PubMed: 20587585]
- 23.
-
Beck RW, Riddlesworth T, Ruedy K, Ahmann A, Bergenstal R, Haller S, Kollman C, Kruger D, McGill JB, Polonsky W, Toschi E, Wolpert H, Price D., DIAMOND Study Group. Effect of Continuous Glucose Monitoring on Glycemic Control in Adults With Type 1 Diabetes Using Insulin Injections: The DIAMOND Randomized Clinical Trial. JAMA. 2017;317(4):371–378. [PubMed: 28118453]
- 24.
-
Lind M, Polonsky W, Hirsch IB, Heise T, Bolinder J, Dahlqvist S, Schwarz E, Ólafsdóttir AF, Frid A, Wedel H, Ahlén E, Nyström T, Hellman J. Continuous Glucose Monitoring vs Conventional Therapy for Glycemic Control in Adults With Type 1 Diabetes Treated With Multiple Daily Insulin Injections: The GOLD Randomized Clinical Trial. JAMA. 2017;317(4):379–387. [PubMed: 28118454]
- 25.
-
Langendam M, Luijf YM, Hooft L, Devries JH, Mudde AH, Scholten RJ. Continuous glucose monitoring systems for type 1 diabetes mellitus. Cochrane Database Syst Rev. 2012;1:CD008101. [PMC free article: PMC6486112] [PubMed: 22258980]
- 26.
-
Yeh HC, Brown TT, Maruthur N, Ranasinghe P, Berger Z, Suh YD, Wilson LM, Haberl EB, Brick J, Bass EB, Golden SH. Comparative effectiveness and safety of methods of insulin delivery and glucose monitoring for diabetes mellitus: a systematic review and meta- analysis. Ann Intern Med. 2012;157(5):336–47. [PubMed: 22777524]
- 27.
-
Pickup JC. The evidence base for diabetes technology: appropriate and inappropriate meta-analysis. J Diabetes Sci Technol. 2013;7(6):1567–74. [PMC free article: PMC3876335] [PubMed: 24351183]
- 28.
-
Pickup JC, Freeman SC, Sutton AJ. Glycaemic control in type 1 diabetes during real time continuous glucose monitoring compared with self monitoring of blood glucose: meta- analysis of randomised controlled trials using individual patient data. BMJ. 2011;343:d3805. [PMC free article: PMC3131116] [PubMed: 21737469]
- 29.
-
Little SA, Leelarathna L, Walkinshaw E, Tan HK, Chapple O, Lubina-Solomon A, Chadwick TJ, Barendse S, Stocken DD, Brennand C, Marshall SM, Wood R, Speight J, Kerr D, Flanagan D, Heller SR, Evans ML, Shaw JA. Recovery of hypoglycemia awareness in long-standing type 1 diabetes: a multicenter 2 × 2 factorial randomized controlled trial comparing insulin pump with multiple daily injections and continuous with conventional glucose self-monitoring (HypoCOMPaSS). Diabetes Care. 2014;37(8):2114–22. [PubMed: 24854041]
- 30.
-
van Beers CA, DeVries JH, Kleijer SJ, Smits MM, Geelhoed-Duijvestijn PH, Kramer MH, Diamant M, Snoek FJ, Serné EH. Continuous glucose monitoring for patients with type 1 diabetes and impaired awareness of hypoglycaemia (IN CONTROL): a randomised, open- label, crossover trial. Lancet Diabetes Endocrinol. 2016;4(11):893–902. [PubMed: 27641781]
- 31.
-
Rubin RR, Peyrot M. STAR 3 Study Group. Health-related quality of life and treatment satisfaction in the Sensor-Augmented Pump Therapy for A1C Reduction 3 (STAR 3) trial. Diabetes Technol Ther. 2012;14(2):143–51. [PMC free article: PMC4845679] [PubMed: 22133037]
- 32.
-
Polonsky WH, Hessler D, Ruedy KJ, Beck RW., DIAMOND Study Group. The Impact of Continuous Glucose Monitoring on Markers of Quality of Life in Adults With Type 1 Diabetes: Further Findings From the DIAMOND Randomized Clinical Trial. Diabetes Care. 2017;40(6):736–741. [PubMed: 28389582]
- 33.
-
Carlson AL, Mullen DM, Bergenstal RM. Clinical Use of Continuous Glucose Monitoring in Adults with Type 2 Diabetes. Diabetes Technol Ther. 2017;19(S2):S4–S11. [PMC free article: PMC5444486] [PubMed: 28541137]
- 34.
-
Vigersky RA, Fonda SJ, Chellappa M, Walker MS, Ehrhardt NM. Short- and long-term effects of real-time continuous glucose monitoring in patients with type 2 diabetes. Diabetes Care. 2012 Jan;35(1):32–8. [PMC free article: PMC3241321] [PubMed: 22100963]
- 35.
-
Beck RW, Riddlesworth TD, Ruedy K, Ahmann A, Haller S, Kruger D, McGill JB, Polonsky W, Price D, Aronoff S, Aronson R, Toschi E, Kollman C, Bergenstal R., DIAMOND Study Group. Continuous Glucose Monitoring Versus Usual Care in Patients With Type 2 Diabetes Receiving Multiple Daily Insulin Injections: A Randomized Trial. Ann Intern Med. 2017 Sep 19;167(6):365–374. [PubMed: 28828487]
- 36.
-
Yeoh E, Choudhary P, Nwokolo M, Ayis S, Amiel SA. Interventions That Restore Awareness of Hypoglycemia in Adults With Type 1 Diabetes: A Systematic Review and Meta-analysis. Diabetes Care. 2015 Aug;38(8):1592–609. [PubMed: 26207053]
- 37.
-
Danne T, Nimri R, Battelino T, Bergenstal RM, Close KL, DeVries JH, Garg S, Heinemann L, Hirsch I, Amiel SA, Beck R, Bosi E, Buckingham B, Cobelli C, Dassau E, Doyle FJ 3rd, Heller S, Hovorka R, Jia W, Jones T, Kordonouri O, Kovatchev B, Kowalski A, Laffel L, Maahs D, Murphy HR, Nørgaard K, Parkin CG, Renard E, Saboo B, Scharf M, Tamborlane WV, Weinzimer SA, Phillip M. International Consensus on Use of Continuous Glucose Monitoring. Diabetes Care. 2017 Dec;40(12):1631–1640. [PMC free article: PMC6467165] [PubMed: 29162583]
- 38.
-
Beck RW, Bergenstal RM, Riddlesworth TD, et al. Validation of time in range as an outcome measure for diabetes clinical trials. Diabetes Care. 2019;42:400–405. [PMC free article: PMC6905478] [PubMed: 30352896]
- 39.
-
Petrie JR, Peters AL, Bergenstal RM, Holl RW, Fleming GA, Heinemann L. Improving the Clinical Value and Utility of CGM Systems: Issues and Recommendations: A Joint Statement of the European Association for the Study of Diabetes and the American Diabetes Association Diabetes Technology Working Group. Diabetes Care. 2017;40(12):1614–1621. [PubMed: 29070577]
- 40.
-
Wallia A, Umpierrez GE, Rushakoff RJ, Klonoff DC, Rubin DJ, Hill Golden S, Cook CB, Thompson B., DTS Continuous Glucose Monitoring in the Hospital Panel. Consensus Statement on Inpatient Use of Continuous Glucose Monitoring. J Diabetes Sci Technol. 2017;11(5):1036–1044. [PMC free article: PMC5950996] [PubMed: 28429611]
- 41.
-
Foster NC, Beck RW, Miller KM, Clements MA, Rickels MR, DiMeglio LA, Maahs DM, Tamborlane WV, Bergenstal R, Smith E, Olson BA, Garg SK. State of Type 1 Diabetes Management and Outcomes from the T1D Exchange in 2016-2018. Diabetes Technol Ther. 2019;21(2):66–72. [PMC free article: PMC7061293] [PubMed: 30657336]
- 42.
-
Tanenbaum ML, Hanes SJ, Miller KM, Naranjo D, Bensen R, Hood KK. Diabetes Device Use in Adults With Type 1 Diabetes: Barriers to Uptake and Potential Intervention Targets. Diabetes Care. 2017;40(2):181–187. [PMC free article: PMC5864141] [PubMed: 27899489]
- 43.
-
Nørgaard K, Scaramuzza A, Bratina N, Lalić NM, Jarosz-Chobot P, Kocsis G, Jasinskiene E, De Block C, Carrette O, Castañeda J, Cohen O., Interpret Study Group. Routine sensor-augmented pump therapy in type 1 diabetes: the INTERPRET study. Diabetes Technol Ther. 2013;15(4):273–80. [PMC free article: PMC3696941] [PubMed: 23438304]
- 44.
-
Juvenile Diabetes Research Foundation Continuous Glucose Monitoring Study Group. Effectiveness of continuous glucose monitoring in a clinical care environment: evidence from the Juvenile Diabetes Research Foundation continuous glucose monitoring (JDRF- CGM) trial. Diabetes Care. 2010;33(1):17–22. [PMC free article: PMC2797966] [PubMed: 19837791]
- 45.
-
DeSalvo DJ, Miller KM, Hermann JM, Maahs DM, Hofer SE, Clements MA, Lilienthal E, Sherr JL, Tauschmann M, Holl RW. T1D Exchange and DPV Registries. Continuous glucose monitoring and glycemic control among youth with type 1 diabetes: International comparison from the T1D Exchange and DPV Initiative. Pediatr Diabetes. 2018;19(7):1271–1275. [PMC free article: PMC6175652] [PubMed: 29923262]
- 46.
- 47.
-
Bay C, Kristensen PL, Pedersen-Bjergaard U, Tarnow L, Thorsteinsson B. Nocturnal continuous glucose monitoring: accuracy and reliability of hypoglycemia detection in patients with type 1 diabetes at high risk of severe hypoglycemia. Diabetes Technol Ther. 2013;15(5):371–7. [PubMed: 23537420]
- 48.
-
Freckmann G, Link M, Westhoff A, Kamecke U, Pleus S, Haug C. Prediction Quality of Glucose Trend Indicators in Two Continuous Tissue Glucose Monitoring Systems. Diabetes Technol Ther. 2018 Aug;20(8):550–556. [PMC free article: PMC6080115] [PubMed: 30067409]
- 49.
-
Jenkins AJ, Krishnamurthy B, Best JD, Cameron FJ, Colman PG, Hamblin PS, O'Connell MA, Rodda C, Teede H, O'Neal DN. An algorithm guiding patient responses to real-time- continuous glucose monitoring improves quality of life. Diabetes Technol Ther. 2011 Feb;13(2):105–9. [PubMed: 21284476]
- 50.
-
Diabetes Research In Children Network (DirecNet) Study Group. Buckingham B, Xing D, Weinzimer S, Fiallo-Scharer R, Kollman C, Mauras N, Tsalikian E, Tamborlane W, Wysocki T, Ruedy K, Beck R. Use of the DirecNet Applied Treatment Algorithm (DATA) for diabetes management with a real-time continuous glucose monitor (the FreeStyle Navigator). Pediatr Diabetes. 2008;9(2):142–7. [PMC free article: PMC2390770] [PubMed: 18221427]
- 51.
-
Scheiner G. Practical CGM: Improving Patient Outcomes Through Continuous Glucose Monitoring. 4th ed. Alexandria, VA: American Diabetes Association; 2015.
- 52.
-
Pettus J, Edelman SV. Recommendations for using real-time continuous glucose monitoring (rtCGM) data for insulin adjustments in type 1 diabetes. J Diabetes Sci Technol. 2017;11(1):138–147. [PMC free article: PMC5375074] [PubMed: 27530720]
- 53.
-
Klonoff DC, Kerr D. A simplified approach using rate of change arrows to adjust insulin with real-time continuous glucose monitoring. J Diabetes Sci Technol. 2017;11(6):1063–1069. [PMC free article: PMC5951054] [PubMed: 28884599]
- 54.
-
Aleppo G, Laffel LM, Ahmann AJ, Hirsch IB, Kruger DF, Peters A, Weinstock RS, Harris DR. A Practical Approach to Using Trend Arrows on the Dexcom G5 CGM System for the Management of Adults with Diabetes. Journal of the Endocrine Society. 2017;1(12):1445–1460. [PMC free article: PMC5760210] [PubMed: 29344577]
- 55.
-
Ajjan RA, Cummings MH, Jennigs P, Leelarathna L, Rayman G, Wilmot E. Optimising use of rate-of-change trend arrows for insulin dosing decisions using the FreeStyle Libre Flash Glucose Monitoring System. Diabetes and Vascular Disease Research. 2018;16(1):3–12. [PubMed: 30175925]
- 56.
-
Gonzales WV, Mobashsher AT, Abbosh A. The Progress of Glucose Monitoring—A Review of Invasive to Minimally and Non-Invasive Techniques, Devices, and Sensors. Sensors. 2019;19(4):800. [PMC free article: PMC6412701] [PubMed: 30781431]
- 57.
-
Aleppo G, Ruedy KJ, Riddlesworth TD, Kruger DF, Peters AL, Hirsch I, Bergenstal RM, Toschi E, Ahmann AJ, Shah VN, Rickels MR, Bode BW, Philis-Tsimikas A, Pop-Busui R, Rodriguez H, Eyth E, Bhargava A, Kollman C, Beck RW., REPLACE-BG Study Group. REPLACE-BG: A Randomized Trial Comparing Continuous Glucose Monitoring With and Without Routine Blood Glucose Monitoring in Adults With Well-Controlled Type 1 Diabetes. Diabetes Care. 2017;40(4):538–545. [PMC free article: PMC5864100] [PubMed: 28209654]
- 58.
- 59.
- 60.
-
Aronson R, Abitbol A, Tweden KS. First assessment of the performance of an implantable continuous glucose monitoring system through 180 days in a primarily adolescent population with type 1 diabetes. Diabetes Obes Metab. 2019;21(7):1689–1694. [PMC free article: PMC6618327] [PubMed: 30938036]
- 61.
-
Kropff J, Choudhary P, Neupane S, Barnard K, Bain SC, Kapitza C, Forst T, Link M, Dehennis A, DeVries JH. Accuracy and Longevity of an Implantable Continuous Glucose Sensor in the PRECISE Study: A 180-Day, Prospective, Multicenter, Pivotal Trial. Diabetes Care. 2017 Jan;40(1):63–68. [PubMed: 27815290]
- 62.
-
Lorenz C, Sandoval W, Mortellaro M. Interference Assessment of Various Endogenous and Exogenous Substances on the Performance of the Eversense Long-Term Implantable Continuous Glucose Monitoring System. Diabetes Technol Ther. 2018;20(5):344–352. [PMC free article: PMC5963543] [PubMed: 29600877]
- 63.
- 64.
-
Deiss D, Szadkowska A, Gordon D, Mallipedhi A, Schütz-Fuhrmann I, Aguilera E, Ringsell C, De Block C, Irace C. Clinical Practice Recommendations on the Routine Use of Eversense, the First Long-Term Implantable Continuous Glucose Monitoring System. Diabetes Technol Ther. 2019;21(5):254–264. [PMC free article: PMC6532544] [PubMed: 31021180]
- 65.
-
Šoupal J, Petruželková L, Flekač M, Pelcl T, Matoulek M, Daňková M, Škrha J, Svačina Š, Prázný M. Comparison of Different Treatment Modalities for Type 1 Diabetes, Including Sensor-Augmented Insulin Regimens, in 52 Weeks of Follow-Up: A COMISAIR Study. Diabetes Technol Ther. 2016 Sep;18(9):532–8. [PMC free article: PMC5035377] [PubMed: 27482825]
- 66.
-
Boughton CK, Hovorka R. Is an artificial pancreas (closed-loop system) for Type 1 diabetes effective? Diabet Med. 2019;36(3):279–286. [PubMed: 30183096]
- 67.
-
Melmer A, Züger T, Lewis DM, Leibrand S, Stettler C, Laimer M. Glycaemic Control in Individuals with Type 1 Diabetes using an artificial open source pancreas system (OpenAPS). Diabetes Obesity and Metabolism. 2019;21(10):2333–2337. [PubMed: 31183929]
- 68.
-
Marshall DC, Holloway M, Korer M, Woodman J, Brackenridge A, Hussain S. Do-It- Yourself Artificial Pancreas Systems in Type 1 Diabetes: Perspectives of Two Adult Users, a Caregiver and Three Physicians. Diabetes Ther. 2019;10(5):1553–1564. [PMC free article: PMC6778569] [PubMed: 31440989]
- 69.
- 70.
- 71.
-
Hernando ME, Garcia-Sáez G, Gómez EJ, Pérez-Gandia C, Rodriguez-Herrero A. Automated Insulin Delivery: The Artificial Pancreas Technical Challenges. American Journal of Therapeutics. 2019:1075–2765. [PubMed: 31567196]
- 72.
-
Bailey TS, Ahmann A, Brazg R, Christiansen M, Garg S, Watkins E, Welsh JB, Lee SW. Accuracy and acceptability of the 6-day Enlite continuous subcutaneous glucose sensor. Diabetes Technol Ther. 2014;16(5):277–83. [PubMed: 24758729]
- 73.
-
Bergenstal RM, Klonoff DC, Garg SK, Bode BW, Meredith M, Slover RH, Ahmann AJ, Welsh JB, Lee SW, Kaufman FR., ASPIRE In-Home Study Group. Threshold-based insulin-pump interruption for reduction of hypoglycemia. N Engl J Med. 2013;369(3):224–32. [PubMed: 23789889]
- 74.
-
Ly TT, Nicholas JA, Retterath A, Lim EM, Davis EA, Jones TW. Effect of sensor- augmented insulin pump therapy and automated insulin suspension vs standard insulin pump therapy on hypoglycemia in patients with type 1 diabetes: a randomized clinical trial. JAMA. 2013;310(12):1240–7. [PubMed: 24065010]
- 75.
-
Abraham MB, Nicholas JA, Smith GJ, Fairchild JM, King BR, Ambler GR, Cameron FJ, Davis EA, Jones TW., PLGM Study Group. Reduction in Hypoglycemia With the Predictive Low-Glucose Management System: A Long-term Randomized Controlled Trial in Adolescents With Type 1 Diabetes. Diabetes Care. 2018;41(2):303–310. [PubMed: 29191844]
- 76.
-
Predictive Low-Glucose Suspend Reduces Hypoglycemia in Adults, Adolescents, and Children With Type 1 Diabetes in an At-Home Randomized Crossover Study: Results of the PROLOG Trial. Forlenza GP, Li Z, Buckingham BA, Pinsker JE, Cengiz E, Wadwa RP, Ekhlaspour L, Church MM, Weinzimer SA, Jost E, Marcal T, Andre C, Carria L, Swanson V, Lum JW, Kollman C, Woodall W, Beck RW. Diabetes Care. 2018;41(10):2155–2161. [PubMed: 30089663]
- 77.
-
Christiansen MP, Garg SK, Brazg R, Bode BW, Bailey TS, Slover RH, Sullivan A, Huang S, Shin J, Lee SW, Kaufman FR. Accuracy of a Fourth-Generation Subcutaneous Continuous Glucose Sensor. Diabetes Technol Ther. 2017;19(8):446–456. [PMC free article: PMC5567873] [PubMed: 28700272]
- 78.
-
Bergenstal RM, Garg S, Weinzimer SA, Buckingham BA, Bode BW, Tamborlane WV, Kaufman FR. Safety of a Hybrid Closed-Loop Insulin Delivery System in Patients With Type 1 Diabetes. JAMA. 2016;316(13):1407–1408. [PubMed: 27629148]
- 79.
-
Lal RA, Basina M, Maahs DM, Hood K, Buckingham B, Wilson DM. One Year Clinical Experience of the First Commercial Hybrid Closed-Loop. Diabetes Care. 2019;42(12):2190–2196. [PMC free article: PMC6868462] [PubMed: 31548247]
- 80.
- 81.
-
Brown SA, Kovatchev BP, Raghinaru D, et al. Six-month randomized, multicenter trial of closed-loop control in type 1 diabetes. N Engl J Med. 2019;381:1707–1717. [PMC free article: PMC7076915] [PubMed: 31618560]
- 82.
-
Tauschmann M, Thabit H, Bally L, Allen JM, Hartnell S, Wilinska ME, Ruan Y, Sibayan J, Kollman C, Cheng P, Beck RW, Acerini CL, Evans ML, Dunger DB, Elleri D, Campbell F, Bergenstal RM, Criego A, Shah VN, Leelarathna L, Hovorka R. Closed-Loop Insulin Delivery in suboptimally Controlled Type 1 Diabetes: A Multicentre, 12-Week Randomised Trial. Lancet. 2018;392(10155):1321–1329. [PMC free article: PMC6182127] [PubMed: 30292578]
- 83.
- 84.
- 85.
-
Bekiari E, Kitsios K, Thabit H, Tauschmann M, Athanasiadou E, Karagiannis T, Haidich AB, Hovorka R, Tsapas A. Artificial pancreas treatment for outpatients with type 1 diabetes: systematic review and meta-analysis. BMJ. 2018;361:k1310. [PMC free article: PMC5902803] [PubMed: 29669716]
- 86.
-
Weisman A, Bai JW, Cardinez M, Kramer CK, Perkins BA. Effect of artificial pancreas systems on glycaemic control in patients with type 1 diabetes: a systematic review and meta-analysis of outpatient randomised controlled trials. Lancet Diabetes Endocrinol. 2017;5:501–512. [PubMed: 28533136]
- 87.
-
Phillip M, Battelino T, Atlas E, Kordonouri O, Bratina N, Miller S, Biester T, Stefanija MA, Muller I, Nimri R, Danne T. Nocturnal glucose control with an artificial pancreas at a diabetes camp. N Engl J Med. 2013;368(9):824–33. [PubMed: 23445093]
- 88.
-
Breton MD, Cherñavvsky DR, Forlenza GP, DeBoer MD, Robic J, Wadwa RP, Messer LH, Kovatchev BP, Maahs DM. Closed-Loop Control During Intense Prolonged Outdoor Exercise in Adolescents With Type 1 Diabetes: The Artificial Pancreas Ski Study. Diabetes Care. 2017;40(12):1644–1650. [PMC free article: PMC5711335] [PubMed: 28855239]
- 89.
-
Brown SA, Breton MD, Anderson SM, Kollar L, Keith-Hynes P, Levy CJ, Lam DW, Levister C, Baysal N, Kudva YC, Basu A, Dadlani V, Hinshaw L, McCrady-Spitzer S, Bruttomesso D, Visentin R, Galasso S, Del Favero S, Leal Y, Boscari F, Avogaro A, Cobelli C, Kovatchev BP. Overnight Closed-Loop Control Improves Glycemic Control in a Multicenter Study of Adults With Type 1 Diabetes. J Clin Endocrinol Metab. 2017;102(10):3674–3682. [PMC free article: PMC5630248] [PubMed: 28666360]
- 90.
-
Russell SJ, El-Khatib FH, Sinha M, Magyar KL, McKeon K, Goergen LG, Balliro C, Hillard MA, Nathan DM, Damiano ER. Outpatient glycemic control with a bionic pancreas in type 1 diabetes. N Engl J Med. 2014;371(4):313–325. [PMC free article: PMC4183762] [PubMed: 24931572]
- 91.
-
El-Khatib FH, Russell SJ, Magyar KL, Sinha M, McKeon K, Nathan DM, Damiano ER. Autonomous and continuous adaptation of a bihormonal bionic pancreas in adults and adolescents with type 1 diabetes. J Clin Endocrinol Metab. 2014;99(5):1701–11. [PMC free article: PMC4010702] [PubMed: 24483160]
- 92.
-
Haidar A, Rabasa-Lhoret R, Legault L, Lovblom LE, Rakheja R, Messier V, D'Aoust É, Falappa CM, Justice T, Orszag A, Tschirhart H, Dallaire M, Ladouceur M, Perkins BA. Single- and Dual-Hormone Artificial Pancreas for Overnight Glucose Control in Type 1 Diabetes. J Clin Endocrinol Metab. 2016;101(1):214–23. [PubMed: 26523526]
- 93.
-
Haidar A, Rabasa-Lhoret R, Legault L, Lovblom LE, Rakheja R, Messier V, D'Aoust É, Falappa CM, Justice T, Orszag A, Tschirhart H, Dallaire M, Ladouceur M, Perkins BA. Single- and Dual-Hormone Artificial Pancreas for Overnight Glucose Control in Type 1 Diabetes. J Clin Endocrinol Metab. 2016;101(1):214–23. [PubMed: 26523526]
- 94.
-
Taleb N, Emami A, Suppere C, Messier V, Legault L, Ladouceur M, Chiasson JL, Haidar A, Rabasa-Lhoret R. Efficacy of single-hormone and dual-hormone artificial pancreas during continuous and interval exercise in adult patients with type 1 diabetes: randomised controlled crossover trial. Diabetologia. 2016;59(12):2561–2571. [PubMed: 27704167]
- 95.
-
Haidar A, Messier V, Legault L, Ladouceur M, Rabasa-Lhoret R. Outpatient 60-hour day- and-night glucose control with dual-hormone artificial pancreas, single-hormone artificial pancreas, or sensor-augmented pump therapy in adults with type 1 diabetes: An open- label, randomised, crossover, controlled trial. Diabetes Obes Metab. 2017;19(5):713–720. [PubMed: 28094472]
- 96.
-
Abitbol A, Rabasa-Lhoret R, Messier V, Legault L. SMaoui M, Cohen N, Haidar A. Overnight Glucose Control with Dual- and Single-Hormone Artificial Pancreas in Type 1 Diabetes with Hypoglycemia Unawareness: A Randomized Controlled Trial. Diabetes Technology Therapeutics. 2018;20(3):189–196. [PubMed: 29393675]
- 97.
-
El-Khatib FH, Balliro C, Hillard MA, Magyar KL, Ekhlaspour L, Sinha M, Mondesir D, Esmaeili A, Hartigan C, Thompson MJ, Malkani S, Lock JP, Harlan DM, Clinton P, Frank E, Wilson DM, DeSalvo D, Norlander L, Ly T, Buckingham BA, Diner J, Dezube M, Young LA, Goley A, Kirkman MS, Buse JB, Zheng H, Selagamsetty RR, Damiano ER, Russell SJ. Home use of a bihormonal bionic pancreas versus insulin pump therapy in adults with type 1 diabetes: a multicentre randomised crossover trial. Lancet. 2017;389(10067):369–380. [PMC free article: PMC5358809] [PubMed: 28007348]
- 98.
-
Bailey TS, Grunberger G, Bode BW, Handelsman Y, Hirsch IB, Jovanovič L, Roberts VL, Rodbard D, Tamborlane WV, Walsh J., American Association of Clinical Endocrinologists (AACE). American College of Endocrinology (ACE). American Association of Clinical Endocrinologists and American College of Endocrinology 2016 Outpatient Glucose Monitoring Consensus Statement. Endocr Pract. 2016;22(2):231–61. [PubMed: 26848630]
- 99.
-
Lin T, Gal A, Mayzel Y, Horman K, Bahartan K. Non-Invasive Glucose Monitoring: A Review of Challenges and Recent Advances. Curr Trends in Biomedical Eng & Biosci. 2017;6(5):555696.
- 100.
-
Cooke D, Hurel SJ, Casbard A, Steed L, Walker S, Meredith S, Nunn AJ, Manca A, Sculpher M, Barnard M, Kerr D, Weaver JU, Ahlquist J, Newman SP. Randomized controlled trial to assess the impact of continuous glucose monitoring on HbA(1c) in insulin-treated diabetes (MITRE Study). Diabet Med. 2009;26(5):540–7. [PubMed: 19646195]
- 101.
- 102.
- 103.
- 104.
-
Pfützner A., Strobl S., Demircik F., Redert L., Pfützner J., Pfützner A.H., Lier A. Evaluation of a New Noninvasive Glucose Monitoring Device by Means of Standardized Meal Experiments. J. Diabetes Sci. Technol. 2018;12(6):1178–83. [PMC free article: PMC6232728] [PubMed: 29451016]
- 105.
- 106.
-
Hadar E., Chen R., Toledano Y., Tenenbaum-Gavish K., Atzmon Y., Hod M. Noninvasive, continuous, real-time glucose measurements compared to reference laboratory venous plasma glucose values. J. Matern. Fetal Neonatal Med. 2019;32(20):3393–3400. [PubMed: 29635953]
- 107.
-
Saha S., Cano-Garcia H., Sotiriou I., Lipscombe O., Gouzouasis I., Koutsoupidou M., Palikaras G., Mackenzie R., Reeve T., Kosmas P., et al. A Glucose Sensing System Based on Transmission Measurements at Millimetre Waves using Micro strip Patch Antennas. Sci. Rep. 2017;7:6855. [PMC free article: PMC5537249] [PubMed: 28761121]
- 108.
- 109.
-
Horman K, Mayzel Y, Gal A, Bahartan K, Drexler A, Lin T. Performance and User Experience Evaluation of a Non-Invasive Glucose Monitoring Device. Int J Diabetes Metab Disord. 2016;1(2):1–7.
- 110.
-
Malanda UL, Welschen LM, Riphagen II, Dekker JM, Nijpels G, Bot SD. Self-monitoring of blood glucose in patients with type 2 diabetes mellitus who are not using insulin. Cochrane Database Syst Rev. 2012;1:CD005060. [PubMed: 22258959]
- 111.
-
Cahn A, Akirov A, Raz I. Digital health technology and diabetes management. J Diabetes. 2018;10(1):10–17. [PubMed: 28872765]
- 112.
-
Dinh-Le C, Chuang R, Chokshi S, Mann D. Wearable Health Technology and Electronic Health Record Integration: Scoping Review and Future Directions. JMIR Mhealth Uhealth. 2019;7(9):e12861. [PMC free article: PMC6746089] [PubMed: 31512582]
- 113.
-
Klonoff DC, Kerr D. Overcoming Barriers to Adoption of Digital Health Tools for Diabetes. J Diabetes Sci Technol. 2018;12(1):3–6. [PMC free article: PMC5761987] [PubMed: 28969460]
- 114.
-
Klonoff DC, Kerr D, Kleidermacher D. Now Is the Time for a Security and Safety Standard for Consumer Smartphones Controlling Diabetes Devices. J Diabetes Sci Technol. 2017;11(5):870–873. [PMC free article: PMC5951005] [PubMed: 28728436]
- 115.
-
Blackwell M, Wheeler BJ. Clinical review: the misreporting of logbook, download, and verbal self-measured blood glucose in adults and children with type I diabetes. Acta Diabetol. 2017;54(1):1–8. [PubMed: 27605000]
- 116.
-
Beck RW. Downloading Diabetes Device Data: Empowering Patients to Download at Home to Achieve Better Outcomes. Diabetes Technol Ther. 2015;17(8):536–7. [PubMed: 26060890]
- 117.
-
Lee JM, Hirschfeld E, Wedding J. A Patient-Designed Do-It-Yourself Mobile Technology System for Diabetes: Promise and Challenges for a New Era in Medicine. JAMA. 2016;315(14):1447–8. [PubMed: 27115262]
- 118.
-
Wong JC, Neinstein AB, Spindler M, Adi S. A minority of patients with type 1 diabetes routinely downloads and retrospectively reviews device data. Diabetes Technol Ther. 2015;17:555–562. [PMC free article: PMC4529086] [PubMed: 26133226]
- 119.
-
Miller KM, Foster NC, Beck RW, Bergenstal RM, DuBose SN, DiMeglio LA, Maahs DM, Tamborlane WV. T1D Exchange Clinic Network. Current state of type 1 diabetes treatment in the U.S.: updated data from the T1D Exchange clinic registry. Diabetes Care. 2015;38(6):971–8. [PubMed: 25998289]
- 120.
- 121.
- 122.
-
Blenner SR, Köllmer M, Rouse AJ, Daneshvar N, Williams C, Andrews LB. Privacy Policies of Android Diabetes Apps and Sharing of Health Information. JAMA. 2016;315:1051–2. [PubMed: 26954415]
- 123.
-
Jacques Rose K, Petrut C, L'Heveder R, de Sabata S. IDF Europe position on mobile applications in diabetes. Diabetes Res Clin Pract. 2019;149:139–46. [PubMed: 28951337]
- 124.
-
Klonoff DC, Kerr D, Wong JC, Pavlovic Y, Koliwad S, Hu J, Salber P, Aguilera A, Long W, Hamilton G, Chen KY, Adi S. Digital Diabetes Congress 2017. J Diabetes Sci Technol. 2017;11(5):1045–1052. [PMC free article: PMC5951004] [PubMed: 28786320]
- 125.
-
Rose KJ, Petrut C, L'Heveder R, de Sabata S. IDF Europe's position on mobile applications in diabetes. Diabetes Res Clin Pract. 2019;149:39–46. [PubMed: 28951337]
- 126.
-
Hou C, Carter B, Hewitt J, Francisa T, Mayor S. Do Mobile Phone Applications Improve Glycemic Control (HbA1c) in the Self-management of Diabetes? A Systematic Review, Meta-analysis, and GRADE of 14 Randomized Trials. Diabetes Care. 2016;39(11):2089–2095. [PubMed: 27926892]
- 127.
-
Cui M, Wu X, Mao J, Wang X, Nie M. T2DM Self-Management via Smartphone Applications: A Systematic Review and Meta-Analysis. PLoS One. 2016;11(11):e0166718. [PMC free article: PMC5115794] [PubMed: 27861583]
- 128.
-
Bonoto BC, de Araújo VE, Godói IP, de Lemos LL, Godman B, Bennie M, Diniz LM, Junior AA. Efficacy of Mobile Apps to Support the Care of Patients With Diabetes Mellitus: A Systematic Review and Meta-Analysis of Randomized Controlled Trials. JMIR Mhealth Uhealth. 2017;5(3):e4. [PMC free article: PMC5352856] [PubMed: 28249834]
- 129.
-
Veazie S, Winchell K, Gilbert J, Paynter R, Ivlev I, Eden K, Nussbaum K, Weiskopf N, Guise JM, Helfand M. Mobile Applications for Self-Management of Diabetes [Internet]. Rockville (MD): Agency for Healthcare Research and Quality (US); 2018 May. [PubMed: 30088878]
- 130.
-
Wu Y, Yao X, Vespasiani G, Nicolucci A, Dong Y, Kwong J, Li L, Sun X, Tian H, Li S. Mobile App-Based Interventions to Support Diabetes Self-Management: A Systematic Review of Randomized Controlled Trials to Identify Functions Associated with Glycemic Efficacy. JMIR Mhealth Uhealth. 2017;5(3):e35. [PMC free article: PMC5373677] [PubMed: 28292740]
- 131.
-
Adu MD, Malabu UH, Callander EJ, Malau-Aduli AE, Malau-Aduli BS. Considerations for the Development of Mobile Phone Apps to Support Diabetes Self-Management: Systematic Review. JMIR Mhealth Uhealth. 2018;6(6):e10115. [PMC free article: PMC6035345] [PubMed: 29929949]
- 132.
-
Martinez-Millana A, Jarones E, Fernandez-Llatas C, Hartvigsen G, Traver V. App Features for Type 1 Diabetes Support and Patient Empowerment: Systematic Literature Review and Benchmark Comparison. JMIR Mhealth Uhealth. 2018;6(11):e12237. [PMC free article: PMC6282013] [PubMed: 30463839]
- 133.
-
Fu H, McMahon SK, Gross CR, Adam TJ, Wyman JF. Usability and clinical efficacy of diabetes mobile applications for adults with type 2 diabetes: A systematic review. Diabetes Res Clin Pract. 2017;131:70–81. [PubMed: 28692830]
- 134.
-
Chavez S, Fedele D, Guo Y, Bernier A, Smith M, Warnick J, Modave F. Mobile Apps for the Management of Diabetes. Diabetes Care. 2017;40(10):e145–e146. [PMC free article: PMC5864023] [PubMed: 28774944]
- 135.
-
Brzan PP, Rotman E, Pajnkihar M, Klanjsek P. Mobile Applications for Control and Self Management of Diabetes: A Systematic Review. J Med Syst. 2016;40(9):210. [PubMed: 27520615]
- 136.
-
Katz LB, Dirani RG, Li G, Randoll RA, Mahoney JJ. Automated glycemic pattern analysis can improve health care professional efficiency and accuracy. J Diabetes Sci Technol. 2013;7(1):163–6. [PMC free article: PMC3692229] [PubMed: 23439173]
- 137.
-
Parkin CG, Davidson JA. Value of self-monitoring blood glucose pattern analysis in improving diabetes outcomes. J Diabetes Sci Technol. 2009;3(3):500–8. [PMC free article: PMC2769875] [PubMed: 20144288]
- 138.
-
Eiland L, McLarney M, Thangavelu T, Drincic A. App-Based Insulin Calculators: Current and Future State. Curr Diab Rep. 2018;18(11):123. [PubMed: 30284645]
- 139.
-
Shashaj B, Busetto E, Sulli N. Benefits of a bolus calculator in pre- and postprandial glycaemic control and meal flexibility of paediatric patients using continuous subcutaneous insulin infusion (CSII). Diabet Med. 2008;25(9):1036–42. [PubMed: 18937673]
- 140.
-
Sussman A, Taylor EJ, Patel M, Ward J, Alva S, Lawrence A, Ng R. Performance of a glucose meter with a built-in automated bolus calculator versus manual bolus calculation in insulin-using subjects. J Diabetes Sci Technol. 2012;6(2):339–44. [PMC free article: PMC3380776] [PubMed: 22538144]
- 141.
-
Maurizi AR, Lauria A, Maggi D, Palermo A, Fioriti E, Manfrini S, Pozzilli P. A novel insulin unit calculator for the management of type 1 diabetes. Diabetes Technol Ther. 2011;13(4):425–8. [PubMed: 21355727]
- 142.
-
Huckvale K, Adomaviciute S, Prieto JT, Leow MK, Car J. Smartphone apps for calculating insulin dose: a systematic assessment. BMC Med. 2015;13(1):106. [PMC free article: PMC4433091] [PubMed: 25943590]
- 143.
-
Hirsch IB, Parkin CG. Unknown Safety and Efficacy of Smartphone Bolus Calculator Apps Puts Patients at Risk for Severe Adverse Outcomes. J Diabetes Sci Technol. 2016;10(4):977–80. [PMC free article: PMC4928215] [PubMed: 26798082]
- 144.
- 145.
-
Barnard K, Parkin C, Young A, Ashraf M. Use of an automated bolus calculator reduces fear of hypoglycemia and improves confidence in dosage accuracy in patients with type 1 diabetes mellitus treated with multiple daily insulin injections. J Diabetes Sci Technol. 2012;6(1):144–149. [PMC free article: PMC3320831] [PubMed: 22401332]
- 146.
-
Ziegler R, Cavan DA, Cranston I, Barnard K, Ryder J, Vogel C, Parkin CG, Koehler W, Vesper I, Petersen B, Schweitzer MA, Wagner RS. Use of an insulin bolus advisor improves glycemic control in multiple daily insulin injection (MDI) therapy patients with suboptimal glycemic control: first results from the ABACUS trial. Diabetes Care. 2013;36(11):3613–9. [PMC free article: PMC3816874] [PubMed: 23900590]
- 147.
-
Sussman A, Taylor EJ, Patel M, Ward J, Alva S, Lawrence A, Ng R. Performance of a glucose meter with a built-in automated bolus calculator versus manual bolus calculation in insulin-using subjects. J Diabetes Sci Technol. 2012;6(2):339–44. [PMC free article: PMC3380776] [PubMed: 22538144]
- 148.
- 149.
-
Charpentier G, Benhamou PY, Dardari D, Clergeot A, Franc S, Schaepelynck-Belicar P, Catargi B, Melki V, Chaillous L, Farret A, Bosson JL, Penfornis A. TeleDiab Study Group. The Diabeo software enabling individualized insulin dose adjustments combined with telemedicine support improves HbA1c in poorly controlled type 1 diabetic patients: a 6- month, randomized, open-label, parallel-group, multicenter trial (TeleDiab 1 Study). Diabetes Care. 2011;34(3):533–9. [PMC free article: PMC3041176] [PubMed: 21266648]
- 150.
-
Rossi MC, Nicolucci A, Di Bartolo P, Bruttomesso D, Girelli A, Ampudia FJ, et al. Diabetes Interactive Diary: a new telemedicine system enabling flexible diet and insulin therapy while improving quality of life: an open-label, international,multicenter, randomized study. Diabetes Care. 2010;33:109–15. [PMC free article: PMC2797954] [PubMed: 19808926]
- 151.
-
Rossi MC, Nicolucci A, Lucisano G, Pellegrini F, Di Bartolo P, Miselli V, et al. Impact of the "Diabetes Interactive Diary" telemedicine system on metabolic control, risk of hypoglycemia, and quality of life: a randomized clinical trial in type 1 diabetes. Diabetes Technol Ther. 2013;15:670–9. [PubMed: 23844569]
- 152.
-
Bergenstal RM, Bashan E, McShane M, Johnson M, Hodish I. Can a tool that automates insulin titration be a key to diabetes management? Diabetes Technol Ther. 2012;14(8):675–82. [PMC free article: PMC3409453] [PubMed: 22568777]
- 153.
- 154.
- 155.
- 156.
- 157.
-
Bailey TS, Stone JY. A novel pen-based Bluetooth-enabled insulin delivery system with insulin dose tracking and advice. Expert Opin Drug Deliv. 2017;14(5):697–703. [PubMed: 28359171]
- 158.
-
Del Prato S, Nicolucci A, Lovagnini-Scher AC, Turco S, Leotta S, Vespasiani G., ELEONOR Study Group. Telecare Provides comparable efficacy to conventional self- monitored blood glucose in patients with type 2 diabetes titrating one injection of insulin glulisine-the ELEONOR study. Diabetes Technol Ther. 2012;14(2):175–82. [PubMed: 22013886]
- 159.
-
Wojcicki JM, Ladyzynski P, Foltynski P. What we can really expect from telemedicine in intensive diabetes treatment: 10 years later. Diabetes Tech Ther. 2013;15(3):260–268. [PubMed: 23343333]
- 160.
-
Tildesley HD, Po MD, Ross SA. Internet blood glucose monitoring systems provide lasting glycemic benefit in type 1 and 2 diabetes: a systematic review. Med Clin North Am. 2015;99(1):17–33. [PubMed: 25456641]
- 161.
-
Montori VM, Helgemoe PK, Guyart GH, Dean DS, Leung TW, Smith SA, Kudva YC. Telecare for patients with type 1 diabetes and inadequate glycemic control. A randomized controlled trial and meta-analysis. Diabetes Care. 2004;27:1088–1094. [PubMed: 15111526]
- 162.
-
Polisena J, Tran K, Cimon K, Huton B, McGill S, Palmer K. Home telehealth for diabetes management: a systematic review and meta-analysis. Diabetes Obes Metab. 2009;11:913–930. [PubMed: 19531058]
- 163.
-
Quinn CC, Shardell MD, Terrin ML, Barr EA, Ballew SH, Gruber-Baldini AL. Cluster- randomized trial of a mobile phone personalized behavioral intervention for blood glucose control. Diabetes Care. 2011;34(9):1934–42. [PMC free article: PMC3161305] [PubMed: 21788632]
- 164.
- 165.
-
Downing J, Bollyky J, Schneider J. Use of a Connected Glucose Meter and Certified Diabetes Educator Coaching to Decrease the Likelihood of Abnormal Blood Glucose Excursions: The Livongo for Diabetes Program. J Med Internet Res. 2017;19(7):e234. [PMC free article: PMC5527250] [PubMed: 28698167]
- 166.
-
Bollyky JB, Bravata D, Yang J, Williamson M, Schneider J. Remote Lifestyle Coaching Plus a Connected Glucose Meter with Certified Diabetes Educator Support Improves Glucose and Weight Loss for People with Type 2 Diabetes. J Diabetes Res. 2018;2018:3961730. [PMC free article: PMC5977036] [PubMed: 29888288]
- 167.
-
Young LA, Buse JB, Weaver MA, Vu MB, Mitchell CM, Blakeney T, Grimm K, Rees J, Niblock F, Donahue KE., Monitor Trial Group. Glucose Self-monitoring in Non-Insulin-Treated Patients With Type 2 Diabetes in Primary Care Settings: A Randomized Trial. JAMA Intern Med. 2017;177(7):920–929. [PMC free article: PMC5818811] [PubMed: 28600913]
- 168.
-
Bode B, Clarke JG, Johnson J. Use of Decision Support Software to Titrate Multiple Daily Injections Yielded Sustained A1c Reductions After 1 Year. J Diabetes Sci Technol. 2018;12(1):124–128. [PMC free article: PMC5761995] [PubMed: 29251061]
- 169.
- 170.
-
Debong F, Mayer H, Kober J. Real-World Assessments of mySugr Mobile Health App. Diabetes Technol Ther. 2019;21(S2):S235–S240. [PubMed: 31169427]
- 171.
-
Osborn CY, van Ginkel JR, Marrero DG, Rodbard D, Huddleston B, Dachis J. One Drop | Mobile on iPhone and Apple Watch: An evaluation of A1c improvement associated with tracking self-care. JMIR mHealth and uHealth. 2017;5(11):e179. [PMC free article: PMC5729227] [PubMed: 29187344]
- 172.
-
Kumar S, Moseson H, Uppal J, Juusola JL. A diabetes mobile app with in-app coaching from a Certified Diabetes Educator reduces A1c for individuals with type 2 diabetes. Diabetes Educ. 2018;44(3):226–236. [PubMed: 29575982]
- 173.
-
Nimri R, Dassau E, Segall T, Muller I, Bratina N, Kordonouri O, Bello R, Biester T, Dovc K, Tenenbaum A, Brener A, Šimunović M, Sakka SD, Nevo Shenker M, Passone CG, Rutigliano I, Tinti D, Bonura C, Caiulo S, Ruszala A, Piccini B, Giri D, Stein R, Rabbone I, Bruzzi P, Omladič JŠ, Steele C, Beccuti G, Yackobovitch-Gavan M, Battelino T, Danne T, Atlas E, Phillip M. Adjusting insulin doses in patients with type 1 diabetes who use insulin pump and continuous glucose monitoring: Variations among countries and physicians. Diabetes Obes Metab. 2018;20(10):2458–2466. [PubMed: 29885025]
- 174.
-
Weatherly J, Kishnani S, Aye T. Challenges with Patient Adoption of Automated Integration of Blood Glucose Meter Data in the Electronic Health Record. Diabetes Technol Ther. 2019;21:671–674. [PMC free article: PMC6812727] [PubMed: 31335195]
- 175.
-
Jung SY, Kim JW, Hwang H, et al. Development of Comprehensive Personal Health Records Integrating Patient-Generated Health Data Directly From Samsung S-Health and Apple Health Apps: Retrospective Cross-Sectional Observational Study. JMIR Mhealth Uhealth. 2019;7:e12691. [PMC free article: PMC6658253] [PubMed: 31140446]
- 176.
-
Gallagher EJ, Le Roith D, Bloomgarden Z. Review of hemoglobin A(1c) in the management of diabetes. Journal of diabetes. 2009;1:9–17. [PubMed: 20923515]
- 177.
-
Little RR, Roberts WL. A review of variant hemoglobins interfering with hemoglobin A1c measurement. Journal of diabetes science and technology. 2009;3:446–51. [PMC free article: PMC2769887] [PubMed: 20144281]
- 178.
- 179.
-
Knaebel J, Irvin BR, Xie CZ. Accuracy and clinical utility of a point-of-care HbA1c testing device. Postgrad Med. 2013;125(3):91–8. [PubMed: 23748510]
- 180.
-
Jeppsson JO, Kobold U, Barr J, et al. Approved IFCC reference method for the measurement of HbA1c in human blood. Clin Chem Lab Med. 2002;40:78–89. [PubMed: 11916276]
- 181.
-
Miedema K. Standardization of HbA1c and Optimal Range of Monitoring. Scand J Clin Lab Invest Suppl. 2005;240:61–72. [PubMed: 16112961]
- 182.
-
Cohen RM, Snieder H, Lindsell CJ, Beyan H, Hawa MI, Blinko S, Edwards R, Spector TD, Leslie RD. Evidence for independent heritability of the glycation gap (glycosylation gap) fraction of HbA1c in nondiabetic twins. Diabetes Care. 2006;29:1739–1743. [PubMed: 16873773]
- 183.
-
Chalew SA, McCarter RJ, Thomas J, Thomson JL, Hempe JM. A comparison of the Glycosylation Gap and Hemoglobin Glycation Index in patients with diabetes. J Diabetes Complications. 2005;19:218–222. [PubMed: 15993356]
- 184.
-
Rodríguez-Segade S, Rodríguez J, García Lopez JM, Casanueva FF, Camina F. Estimation of the glycation gap in diabetic patients with stable glycemic control. Diabetes Care. 2012;35:2447–2450. [PMC free article: PMC3507558] [PubMed: 22961579]
- 185.
-
Nayak AU, Holland MR, Macdonald DR, Nevill A, Singh BM. Evidence for consistency of the glycation gap in diabetes. Diabetes Care. 2011;34(8):1712–6. [PMC free article: PMC3142043] [PubMed: 21715524]
- 186.
-
Sacks DB, Nathan DM, Lachin JM. Gaps in the glycation gap hypothesis. Clin Chem. 2011;57:150–152. [PubMed: 21127149]
- 187.
-
Kilpatrick ES, Rigby AS, Atkin SL. Variability in the Relationship between Mean Plasma Glucose and HbA1c: Implications for the Assessment of Glycemic Control. Clin Chem. 2007;53(5):897–901. [PubMed: 17384010]
- 188.
-
Quagliaro L, Piconi L, Assaloni R, Martinelli L, Motz E, Ceriello A. Intermittent high glucose enhances apoptosis related to oxidative stress in human umbilical vein endothelial cells: the role of protein kinase C and NAD(P)H-oxidase activation. Diabetes. 2003;52(11):2795–2804. [PubMed: 14578299]
- 189.
-
Inhat M, Green D, Ross K, et al. Reduced antioxidant response of retinal and endothelial cells in response to chronic oscillating glucose levels. Diabetes. 2005;54 Suppl. 1:2314A.
- 190.
- 191.
-
Parrinello CM, Selvin E. Beyond HbA1c and glucose: the role of nontraditional glycemic markers in diabetes diagnosis, prognosis, and management. Curr Diab Rep. 2014;14(11):548. [PMC free article: PMC4214073] [PubMed: 25249070]
- 192.
-
Danese E, Montagnana M, Nouvenne A, Lippi G. Advantages and pitfalls of fructosamine and glycated albumin in the diagnosis and treatment of diabetes. J Diabetes Sci Technol. 2015;9(2):169–76. [PMC free article: PMC4604592] [PubMed: 25591856]
- 193.
-
Herman WH, Cohen RM. Racial and ethnic differences in the relationship between HbA1c and blood glucose: implications for the diagnosis of diabetes. J Clin Endocrinol Metab. 2012;97(4):1067–72. [PMC free article: PMC3319188] [PubMed: 22238408]
- 194.
-
Bergenstal RM, Gal RL, Connor CG, Gubitosi-Klug R, Kruger D, Olson BA, Willi SM, Aleppo G, Weinstock RS, Wood J, Rickels M, DiMeglio LA, Bethin KE, Marcovina S, Tassopoulos A, Lee S, Massaro E, Bzdick S, Ichihara B, Markmann E, McGuigan P, Woerner S, Ecker M, Beck RW. T1D Exchange Racial Differences Study Group. Racial Differences in the Relationship of Glucose Concentrations and Hemoglobin A1c Levels. Ann Intern Med. 2017;167(2):95–102. [PubMed: 28605777]
- 195.
-
Bower JK, Brancati FL, Selvin E. No ethnic differences in the association of glycated hemoglobin with retinopathy: the national health and nutrition examination survey 2005- 2008. Diabetes Care. 2013;36(3):569–73. [PMC free article: PMC3579340] [PubMed: 23069841]
- 196.
-
Parrinello CM, Sharrett AR, Maruthur NM, Bergenstal RM, Grams ME, Coresh J, Selvin E. Racial Differences in and Prognostic Value of Biomarkers of Hyperglycemia. Diabetes Care. 2016;39(4):589–95. [PMC free article: PMC4806772] [PubMed: 26681712]
- 197.
-
Lindsey CC, Carter AW, Mangum S, Greene D, Richardson A, Brown SJ, Essary JL, McCandless B. A prospective, randomized, multicentered controlled trial to compare the annual glycemic and quality outcomes of patients with diabetes mellitus monitored with weekly fructosamine testing versus usual care. Diabetes Technol Ther. 2004;6(3):370–7. [PubMed: 15198841]
- 198.
-
Edelman SV, Bell JM, Serrano RB, Kelemen D. Home testing of fructosamine improves glycemic control in patients with diabetes. Endocr Pract. 2001;7(6):454–8. [PubMed: 11747282]
- 199.
-
Shohat N, Tarabichi M, Tischler EH, Jabbour S, Parvizi J. Serum Fructosamine: A Simple and Inexpensive Test for Assessing Preoperative Glycemic Control. J Bone Joint Surg Am. 2017;99(22):1900–1907. [PubMed: 29135663]
- 200.
-
Selvin E, Rawlings AM, Grams M, Klein R, Sharrett AR, Steffes M, Coresh J. Fructosamine and glycated albumin for risk stratification and prediction of incident diabetes and microvascular complications: a prospective cohort analysis of the Atherosclerosis Risk in Communities (ARIC) study. Lancet Diabetes Endocrinol. 2014;2(4):279–88. [PMC free article: PMC4212648] [PubMed: 24703046]
- 201.
-
Jung M, Warren B, Grams M, Kwong YD, Shafi T, Coresh J, Rebholz CM, Selvin E. Performance of non-traditional hyperglycemia biomarkers by chronic kidney disease status in older adults with diabetes: Results from the Atherosclerosis Risk in Communities Study. J Diabetes. 2018;10(4):276–285. [PMC free article: PMC5867205] [PubMed: 29055090]
- 202.
-
Selvin E, Rawlings AM, Lutsey PL, Maruthur N, Pankow JS, Steffes M, Coresh J. Fructosamine and Glycated Albumin and the Risk of Cardiovascular Outcomes and Death. Circulation. 2015;132(4):269–77. [PMC free article: PMC4612521] [PubMed: 26022911]
- 203.
-
Nathan DM, McGee P, Steffes MW, Lachin JM., DCCT/EDIC Research Group. Relationship of glycated albumin to blood glucose and HbA1c values and to retinopathy, nephropathy, and cardiovascular outcomes in the DCCT/EDIC study. Diabetes. 2014;63(1):282–90. [PMC free article: PMC3868040] [PubMed: 23990364]
- 204.
-
Zhu J, Chen Y, Li C, Tao M, Teng Y. The diagnostic value of glycated albumin in gestational diabetes mellitus. J Endocrinol Invest. 2018;41(1):121–128. [PMC free article: PMC5754373] [PubMed: 28589381]
- 205.
-
Shimizu I, Hiramatsu Y, Omori Y, Nakabayashi M. Comparison of HbA1c and glycated albumin as a control marker for newborn complications in diabetic women in a multicentre study in Japan (Japan glycated albumin study group: study 2). Ann Clin Biochem. 2018;55(6):639–646. [PubMed: 29471686]
- 206.
-
Dungan KM, Buse JB, Largay J, Kelly MM, Button EA, Kato S, Wittlin S. 1,5- anhydroglucitol and postprandial hyperglycemia as measured by continuous glucose monitoring system in moderately controlled patients with diabetes. Diabetes Care. 2006;29:1214–1219. [PubMed: 16731998]
- 207.
-
Lee AK, Lee CJ, Huang ES, Sharrett AR, Coresh J, Selvin E. Risk Factors for Severe Hypoglycemia in Black and White Adults With Diabetes: The Atherosclerosis Risk in Communities (ARIC) Study. Diabetes Care. 2017;40(12):1661–1667. [PMC free article: PMC5711330] [PubMed: 28928117]
- 208.
-
Rebholz CM, Grams ME, Chen Y, Gross AL, Sang Y, Coresh J, Selvin E. Serum Levels of 1,5-Anhydroglucitol and Risk of Incident End-Stage Renal Disease. Am J Epidemiol. 2017;186(8):952–960. [PMC free article: PMC5860021] [PubMed: 28535187]
- 209.
-
Rawlings AM, Sharrett AR, Mosley TH, Ballew SH, Deal JA, Selvin E. Glucose Peaks and the Risk of Dementia and 20-Year Cognitive Decline. Diabetes Care. 2017;40(7):879–886. [PMC free article: PMC5481977] [PubMed: 28500217]
- 210.
-
Selvin E, Rawlings A, Lutsey P, Maruthur N, Pankow JS, Steffes M, Coresh J. Association of 1,5-Anhydroglucitol With Cardiovascular Disease and Mortality. Diabetes. 2016;65(1):201–8. [PMC free article: PMC4686946] [PubMed: 26395741]
Computer Blood Sugar and Tracking A1c Software
Source: https://www.ncbi.nlm.nih.gov/books/NBK279046/
0 Response to "Computer Blood Sugar and Tracking A1c Software"
Post a Comment